A Non-Gaussian Process Monitoring Method Based on Known Data Regression
A known data and process monitoring technology, applied in the field of data-driven process monitoring, can solve the problem of estimated values generated by data models, achieve the effect of accurate normal areas and improve fault detection capabilities
- Summary
- Abstract
- Description
- Claims
- Application Information
AI Technical Summary
Problems solved by technology
Method used
Image
Examples
Embodiment Construction
[0071] The method of the present invention will be described in detail below in conjunction with the accompanying drawings and specific examples of implementation.
[0072] Such as figure 1 As shown, the present invention discloses a non-Gaussian process monitoring method based on known data regression. The specific implementation process of the method of the present invention and its superiority over existing methods will be described below in conjunction with an example of a specific industrial process.
[0073] Design a non-Gaussian process with 5 measured variables according to the following formula:
[0074]
[0075] Among them, the source signal s=[s 1 ,s 2 ] is generated according to the formula shown below:
[0076]
[0077] In the above two formulas (19) and (20), the measurement noise v follows a Gaussian distribution with a mean of 0 and a standard deviation of 0.2, t 1 with t 2Both are random numbers uniformly distributed on the interval [0, 1]. Accord...
PUM
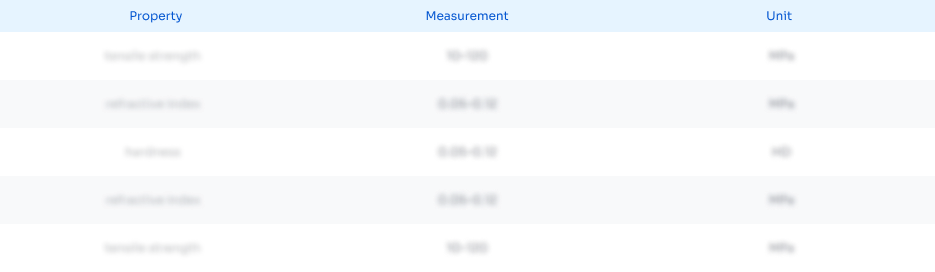
Abstract
Description
Claims
Application Information
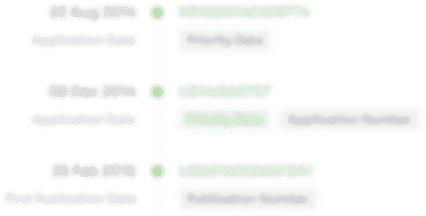
- R&D Engineer
- R&D Manager
- IP Professional
- Industry Leading Data Capabilities
- Powerful AI technology
- Patent DNA Extraction
Browse by: Latest US Patents, China's latest patents, Technical Efficacy Thesaurus, Application Domain, Technology Topic, Popular Technical Reports.
© 2024 PatSnap. All rights reserved.Legal|Privacy policy|Modern Slavery Act Transparency Statement|Sitemap|About US| Contact US: help@patsnap.com