Improved type principal component analysis model-based fault detection method
A technology of fault detection and principal component analysis, which is applied in character and pattern recognition, instruments, computer components, etc., can solve problems such as missing distance correlation feature mining, and achieve good fault detection results
- Summary
- Abstract
- Description
- Claims
- Application Information
AI Technical Summary
Problems solved by technology
Method used
Image
Examples
Embodiment Construction
[0027] The method of the present invention will be described in detail below in conjunction with the accompanying drawings.
[0028] Such as figure 1 As shown, the method of the present invention discloses a fault detection method based on the improved principal component analysis model, and the specific implementation steps are as follows:
[0029] Step 1: Collect data samples under the normal operating state of the production process to form a training data set X∈R n×m , and standardize each variable to get a new data matrix with mean 0 and standard deviation 1 Among them, n is the number of training samples, m is the number of process measurement variables, R is the set of real numbers, and R n×m Represents an n×m-dimensional real number matrix, is the standardized data of the i-th measurement variable, i=1, 2, . . . , m is the subscript of the i-th measurement variable.
[0030] Step 2: Follow the formula Calculate the angular correlation matrix S of the training d...
PUM
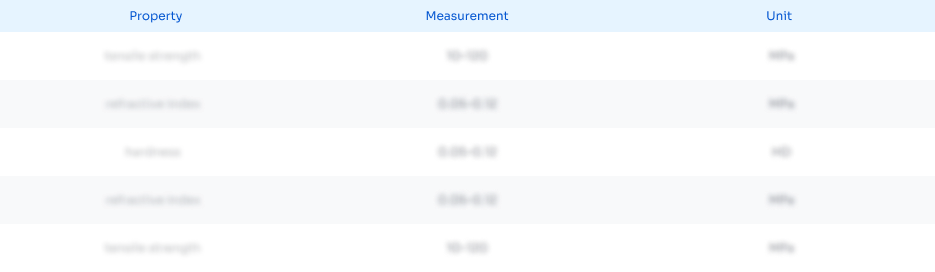
Abstract
Description
Claims
Application Information
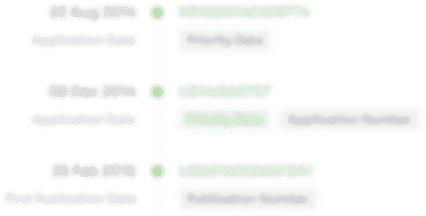
- R&D
- Intellectual Property
- Life Sciences
- Materials
- Tech Scout
- Unparalleled Data Quality
- Higher Quality Content
- 60% Fewer Hallucinations
Browse by: Latest US Patents, China's latest patents, Technical Efficacy Thesaurus, Application Domain, Technology Topic, Popular Technical Reports.
© 2025 PatSnap. All rights reserved.Legal|Privacy policy|Modern Slavery Act Transparency Statement|Sitemap|About US| Contact US: help@patsnap.com