A Supervised Learning-Based Deep Autoencoder for Road Segmentation
An automatic encoder and supervised learning technology, applied in the field of computer vision, can solve problems such as low segmentation accuracy, complex network structure, and poor real-time performance, and achieve simple model, low training time and running time, and good road segmentation effect Effect
- Summary
- Abstract
- Description
- Claims
- Application Information
AI Technical Summary
Problems solved by technology
Method used
Image
Examples
Embodiment Construction
[0022] The present invention proposes a road segmentation method based on a supervised learning deep autoencoder. Firstly, a supervisory layer is added to the traditional autoencoder model, and a known segmentation map of the road environment image is used as supervision information to design an effective Supervised single-layer autoencoders. Then, because the deep network has more abstract and more diversified feature expression capabilities, the present invention establishes a supervised deep autoencoder model to extract deep features of road environment segmentation, and studies the multi-layer of supervised autoencoder In a stacked manner, a supervised deep autoencoder model is trained using existing training samples and their road environment segmentation maps. Finally, the test sample is loaded to obtain its semantic segmentation map, and the drivable road area of the road environment image is determined by the basic method of image processing. Such as figure 1 Shown...
PUM
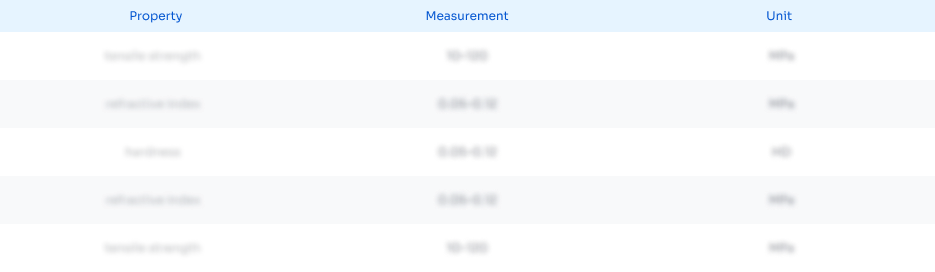
Abstract
Description
Claims
Application Information
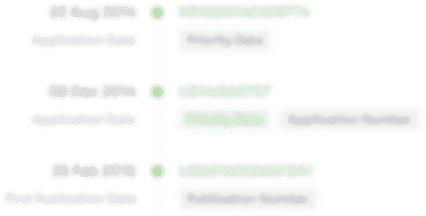
- R&D Engineer
- R&D Manager
- IP Professional
- Industry Leading Data Capabilities
- Powerful AI technology
- Patent DNA Extraction
Browse by: Latest US Patents, China's latest patents, Technical Efficacy Thesaurus, Application Domain, Technology Topic, Popular Technical Reports.
© 2024 PatSnap. All rights reserved.Legal|Privacy policy|Modern Slavery Act Transparency Statement|Sitemap|About US| Contact US: help@patsnap.com