A Fully Convolutional Neural Network and Corresponding Mesostructure Recognition Method
A convolutional neural network and network technology, which is applied in the field of mesoscopic structure recognition of ceramic matrix composite material preforms, can solve the problems of large memory usage and long training time of full convolutional neural network, reduce time and improve accuracy. , The effect of simplifying the recognition operation
- Summary
- Abstract
- Description
- Claims
- Application Information
AI Technical Summary
Problems solved by technology
Method used
Image
Examples
Embodiment Construction
[0042] The present invention is described in further detail now in conjunction with accompanying drawing.
[0043] Such as figure 1 The CMCs_Net full convolutional neural network shown is composed of an encoder network, a decoder network and a classification layer. The convolution kernels in the full convolutional neural network are all 3x3, and the padding operation is performed. The padding size is 1 , to ensure that the size of the feature remains unchanged during the image convolution process.
[0044] The encoder network part consists of five encoders, which are sequentially recorded as encoder one, encoder two, encoder three, encoder four, and encoder five. Each encoder is composed of multiple convolutional layers and a layer of pooling layer in turn. The convolutional layer is used to extract the features of the picture; the pooling layer is used to reduce the resolution of the picture, and the pooling kernel is 2*2, that is The length and width are respectively reduc...
PUM
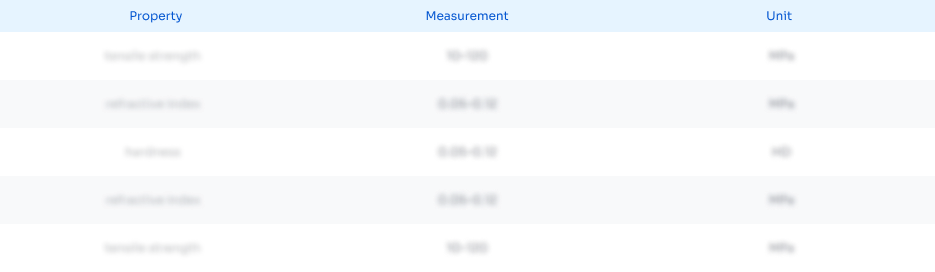
Abstract
Description
Claims
Application Information
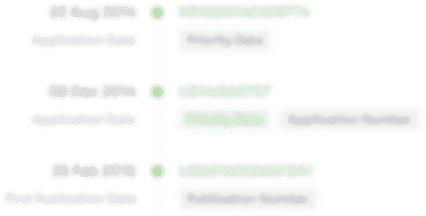
- R&D Engineer
- R&D Manager
- IP Professional
- Industry Leading Data Capabilities
- Powerful AI technology
- Patent DNA Extraction
Browse by: Latest US Patents, China's latest patents, Technical Efficacy Thesaurus, Application Domain, Technology Topic, Popular Technical Reports.
© 2024 PatSnap. All rights reserved.Legal|Privacy policy|Modern Slavery Act Transparency Statement|Sitemap|About US| Contact US: help@patsnap.com