Compact visual descriptor deep neural network generation model in visual retrieval
A deep neural network and generative model technology, which is applied in the field of compact visual descriptor deep neural network generative models, can solve problems such as inability to adapt to application scenarios with limited computing resources and storage resources
- Summary
- Abstract
- Description
- Claims
- Application Information
AI Technical Summary
Problems solved by technology
Method used
Image
Examples
Embodiment Construction
[0052] The following embodiments will describe the present invention in detail with reference to the accompanying drawings.
[0053] The present invention comprises the following steps:
[0054] 1) For the images in the image library, randomly select a part of the images as the training set, and extract the corresponding image local features;
[0055] 2) Randomly combine image local feature set pairs for offline training of the deep neural network model for the training set;
[0056] 3) Train the deep neural network model with the backpropagation algorithm;
[0057] The specific method of training the deep neural network model by the backpropagation algorithm is:
[0058] a) For each batch in the image local feature set pair:
[0059] b) Each batch of local feature sets is input to the deep neural network model, and the gradient value of all parameters of the model is calculated using the back propagation algorithm;
[0060] c) Update model parameters;
[0061] d) exit th...
PUM
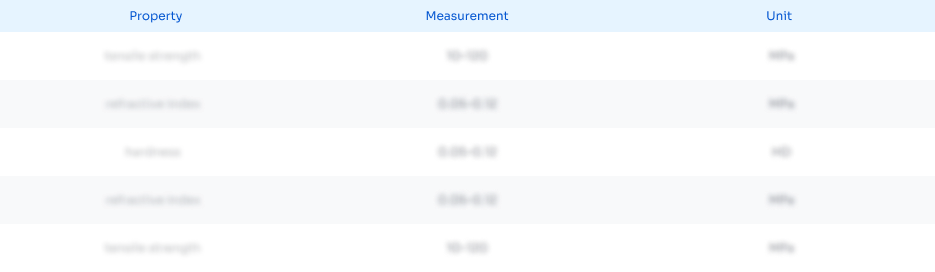
Abstract
Description
Claims
Application Information
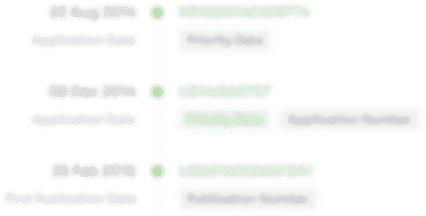
- R&D
- Intellectual Property
- Life Sciences
- Materials
- Tech Scout
- Unparalleled Data Quality
- Higher Quality Content
- 60% Fewer Hallucinations
Browse by: Latest US Patents, China's latest patents, Technical Efficacy Thesaurus, Application Domain, Technology Topic, Popular Technical Reports.
© 2025 PatSnap. All rights reserved.Legal|Privacy policy|Modern Slavery Act Transparency Statement|Sitemap|About US| Contact US: help@patsnap.com