Network driving environment integrated perception model based on convolutional and hollow convolutional structure
A technology that integrates the network and the driving environment. It is applied in the field of advanced automotive driver assistance. It can solve the problems of large amount of calculation, high cost, and repeated calculations, and achieve low computing costs and data labeling costs, improve accuracy, and simple labeling. Effect
- Summary
- Abstract
- Description
- Claims
- Application Information
AI Technical Summary
Problems solved by technology
Method used
Image
Examples
Embodiment Construction
[0023] Below in conjunction with accompanying drawing and specific embodiment the present invention is described in further detail:
[0024] The present invention provides a fusion network driving environment perception model based on convolution and atrous convolution structure, which solves the problem that the current driving environment perception model has a large amount of calculation, many repeated calculations, a single task model solves a single problem, and the semantic segmentation model does not affect the semantic segmentation data set. The requirements are too high (the cost of pixel-level data labeling is too high), and the problem of multi-tasking driving environment perception cannot be completed at the same time.
[0025] A fusion network driving environment perception model based on convolution and hole convolution of the present invention comprises the following steps:
[0026] 1) Capture images of the current driving environment through a camera installed ...
PUM
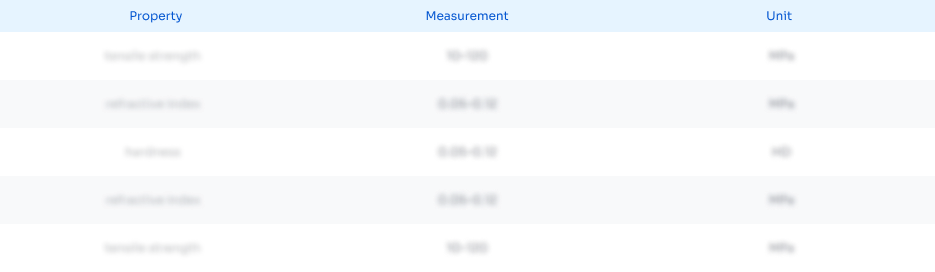
Abstract
Description
Claims
Application Information
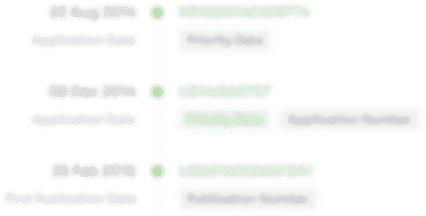
- R&D
- Intellectual Property
- Life Sciences
- Materials
- Tech Scout
- Unparalleled Data Quality
- Higher Quality Content
- 60% Fewer Hallucinations
Browse by: Latest US Patents, China's latest patents, Technical Efficacy Thesaurus, Application Domain, Technology Topic, Popular Technical Reports.
© 2025 PatSnap. All rights reserved.Legal|Privacy policy|Modern Slavery Act Transparency Statement|Sitemap|About US| Contact US: help@patsnap.com