Dynamic Video Segmentation Method Based on Weighted Non-convex Regularization and Iteratively Reconstrained Low-Rank Representation
A low-rank representation and video segmentation technology, applied in character and pattern recognition, instrumentation, computing, etc., can solve problems such as poor anti-noise ability, complex calculation of high-dimensional data, and poor real-time performance
- Summary
- Abstract
- Description
- Claims
- Application Information
AI Technical Summary
Problems solved by technology
Method used
Image
Examples
Embodiment Construction
[0020] The technical solution of the present invention will be further described below in conjunction with the accompanying drawings.
[0021] A dynamic video segmentation method based on weighted non-convex regularization and iteratively re-constrained low-rank representation, including the following steps:
[0022] Step 1, weighted feature learning for error penalty, determines the weight matrix. In reality, the noise is complex, and the distribution of the residual E=X-XZ is far from conventional distributions such as Laplace distribution or Gaussian distribution. Therefore, this method introduces a weight factor to adapt the error term:
[0023]
[0024] in is a data matrix with n samples as its columns, Z is an expression matrix, ||·|| F is the Frobenius norm constraint, that is, the square root of the sum of the squares of all elements, and ⊙ represents the multiplication symbol of the elements.
[0025] Considering the large uncertainty of the actual noise point...
PUM
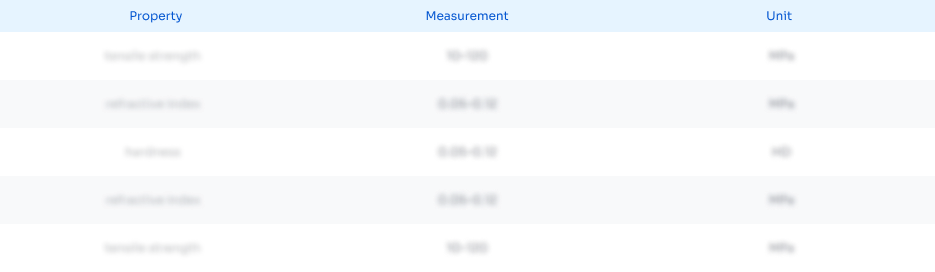
Abstract
Description
Claims
Application Information
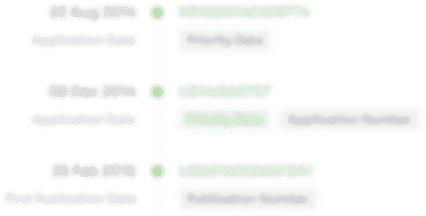
- R&D
- Intellectual Property
- Life Sciences
- Materials
- Tech Scout
- Unparalleled Data Quality
- Higher Quality Content
- 60% Fewer Hallucinations
Browse by: Latest US Patents, China's latest patents, Technical Efficacy Thesaurus, Application Domain, Technology Topic, Popular Technical Reports.
© 2025 PatSnap. All rights reserved.Legal|Privacy policy|Modern Slavery Act Transparency Statement|Sitemap|About US| Contact US: help@patsnap.com