An automatic image annotation method for weakly supervised semantic segmentation
A semantic segmentation and automatic image technology, applied in the field of automatic image annotation of weakly supervised semantic segmentation, can solve problems such as time-consuming and laborious, difficult to achieve accuracy, and machine prediction errors
- Summary
- Abstract
- Description
- Claims
- Application Information
AI Technical Summary
Problems solved by technology
Method used
Image
Examples
specific Embodiment approach
[0069] The invention discloses an automatic image labeling method for weakly supervised semantic segmentation, which combines deep learning-based target detection technology with traditional image segmentation technology to solve the existing problem of manual image labeling of training samples. The purpose of target detection is to locate the bounding box of the target in the video and give semantic labels. For semantic segmentation, the target frame and semantic label can be used as a weakly supervised semantic annotation. Under this weakly supervised image-level semantic annotation, traditional image segmentation techniques are used to construct the association between image-level semantic annotation and pixels. Dense pixel-level classification of objects and backgrounds in a scene infers segmentation templates for image semantic segmentation. figure 1 An emoticon that automatically generates annotated images is given. The target detection gives the frame of the target in ...
PUM
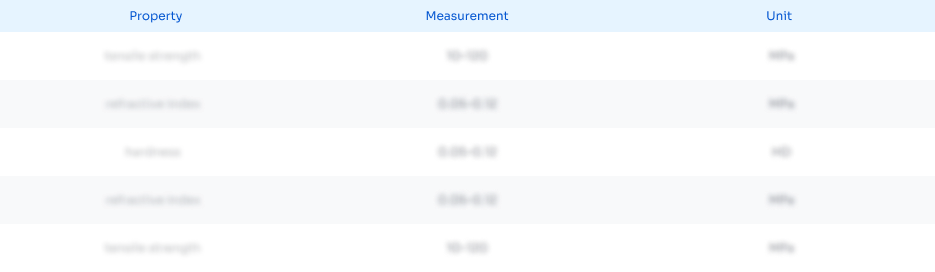
Abstract
Description
Claims
Application Information
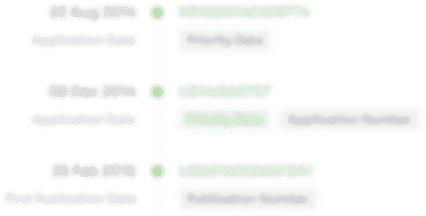
- R&D
- Intellectual Property
- Life Sciences
- Materials
- Tech Scout
- Unparalleled Data Quality
- Higher Quality Content
- 60% Fewer Hallucinations
Browse by: Latest US Patents, China's latest patents, Technical Efficacy Thesaurus, Application Domain, Technology Topic, Popular Technical Reports.
© 2025 PatSnap. All rights reserved.Legal|Privacy policy|Modern Slavery Act Transparency Statement|Sitemap|About US| Contact US: help@patsnap.com