A target classification and recognition method based on multi-source domain fusion transfer learning
A technology of transfer learning and target classification, which is applied in character and pattern recognition, instruments, computer parts, etc., can solve the problem of insufficient accuracy of transfer learning in the single-source field, and achieve a reasonable evaluation reliability effect
- Summary
- Abstract
- Description
- Claims
- Application Information
AI Technical Summary
Problems solved by technology
Method used
Image
Examples
Embodiment 1
[0067] Example 1: Assume that the number of target sample categories to be identified is 3, that is, the identification framework is Ω={ω 1 ,ω 2 ,ω 3}, there are three source domains and one target domain, and the initial classification results are obtained as three evidences (the first classification results) through the classic transfer learning algorithm, and the trust assignment of each evidence is taken as
[0068] m 1 (ω 1 )=0.5,m2 (ω 2 )=0.3,m 3 (ω3) = 0.2;
[0069] m 2 (ω 1 )=0.6,m 2 (ω 2 )=0.2,m(ω 3 ) = 0.2;
[0070] m 3 (ω 1 )=0.2,m 3 (ω 2 )=0.5,m 3 (ω 3 ) = 0.3;
[0071] For the convenience of illustration, the distances calculated before and after mapping are directly given as
[0072]
[0073]
[0074] According to the above two distances, the final distance is The discount coefficient (reliability of the first classification result) obtained from the estimated distance is
[0075] beta 1 =1,β 2 =0.6774,β 3 = 0.6862. The weighted fu...
Embodiment 2
[0078] Embodiment 2: Assume that the basic trust assignment (first classification result) obtained by transfer learning is
[0079] m 1 (ω 1 )=0.7,m 2 (ω 2 )=0.1,m 3 (ω 3 ) = 0.2;
[0080] m 2 (ω 1 )=0.2,m 2 (ω 2 )=0.2,m(ω 3 ) = 0.6;
[0081] m 3 (ω 1 )=0.2,m 3 (ω 2 )=0.5,m 3 (ω 3 ) = 0.3;
[0082] The rest of the parameters are the same as mentioned in Example 1. It can be seen from the observation that the three evidences at this time respectively support ω 1 ,ω 3 ,ω 2 , if only one source field is considered, one of the three evidences will be obtained. However, the categories supported by these three evidences are different. Obviously, when there is only one evidence, the classification results obtained are prone to errors, that is, the classification results of a single source field There is a high chance of error. Therefore, the trust assignment (final classification result) obtained by weighted fusion method is
[0083] m(ω 1 )=0.5774, m(ω 2 )=...
PUM
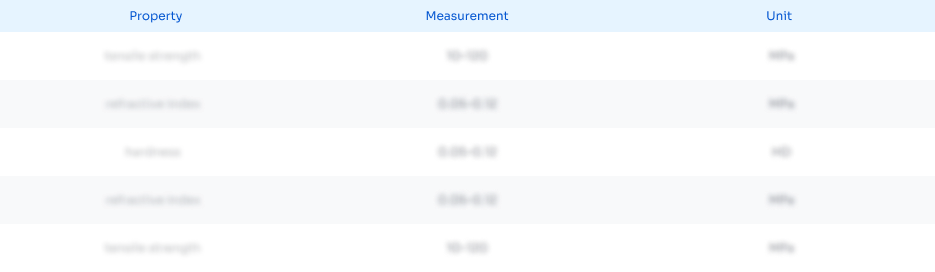
Abstract
Description
Claims
Application Information
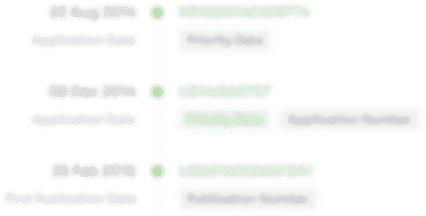
- R&D Engineer
- R&D Manager
- IP Professional
- Industry Leading Data Capabilities
- Powerful AI technology
- Patent DNA Extraction
Browse by: Latest US Patents, China's latest patents, Technical Efficacy Thesaurus, Application Domain, Technology Topic.
© 2024 PatSnap. All rights reserved.Legal|Privacy policy|Modern Slavery Act Transparency Statement|Sitemap