A Channel Information Compression Feedback Method Based on Deep Recurrent Neural Network
A technology of cyclic neural network and channel information, which is applied in the field of channel information compression feedback based on deep cyclic neural network, can solve the problems of unconsidered time redundancy and low feedback accuracy, achieve high-efficiency channel compression feedback, simple implementation process, and improved efficiency effect
- Summary
- Abstract
- Description
- Claims
- Application Information
AI Technical Summary
Problems solved by technology
Method used
Image
Examples
Embodiment Construction
[0061] The technical solutions provided by the present invention will be described in detail below in conjunction with specific examples. It should be understood that the following specific embodiments are only used to illustrate the present invention and are not intended to limit the scope of the present invention.
[0062] The present invention is based on figure 1 The neural network shown is realized. The network is composed of four modules, which are channel information extraction module, channel feature compression module, channel feature decompression module and channel information recovery module. The channel information extraction module includes a convolution layer including a 3×3×2×2 convolution kernel, and a dimension reconstruction unit, which is used to convert the information output by the convolution layer into a one-dimensional tensor. The channel feature compression module includes a parallel fully connected neural network and a long-short-term memory network,...
PUM
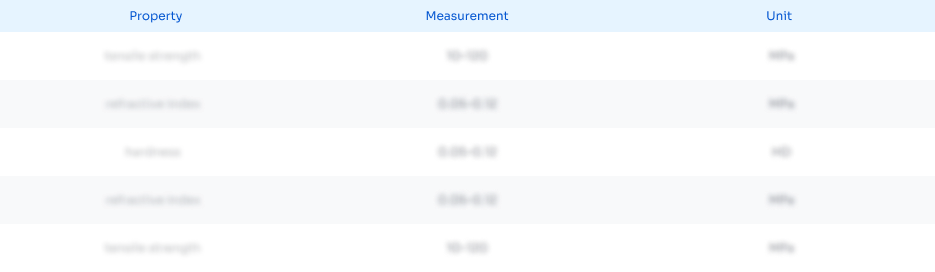
Abstract
Description
Claims
Application Information
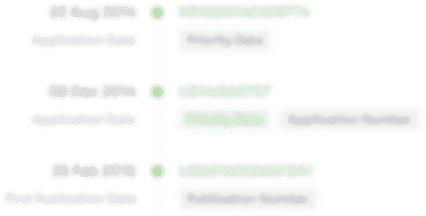
- R&D Engineer
- R&D Manager
- IP Professional
- Industry Leading Data Capabilities
- Powerful AI technology
- Patent DNA Extraction
Browse by: Latest US Patents, China's latest patents, Technical Efficacy Thesaurus, Application Domain, Technology Topic, Popular Technical Reports.
© 2024 PatSnap. All rights reserved.Legal|Privacy policy|Modern Slavery Act Transparency Statement|Sitemap|About US| Contact US: help@patsnap.com