A group abnormal behavior detection method based on deep structure learning
A detection method and anomaly technology, applied in the field of computer information, can solve problems such as difficult acquisition, no effect of multi-abnormal groups, and easy tampering of attribute information by fraudsters, etc., to achieve good results
- Summary
- Abstract
- Description
- Claims
- Application Information
AI Technical Summary
Problems solved by technology
Method used
Image
Examples
Embodiment Construction
[0035] In order to make the above-mentioned features and advantages of the present invention more comprehensible, the following specific embodiments are described in detail in conjunction with the accompanying drawings.
[0036] The invention utilizes a deep neural network to complete the embedding of a bipartite graph network, and in combination with a density-based clustering method, proposes a group abnormal behavior detection method based on deep structure learning.
[0037] The realistic assumption that the present invention is based on is: fraudsters will comment on target products as much as possible, while ordinary users will not comment too much on these products, and the present invention hopes to detect All anomalous groups that contain groups of fraudsters and corresponding target items.
[0038] Such as image 3 As shown, the specific solution idea of the present invention is: for a given bipartite graph G=(U, V, E), firstly, the source nodes and sink nodes in ...
PUM
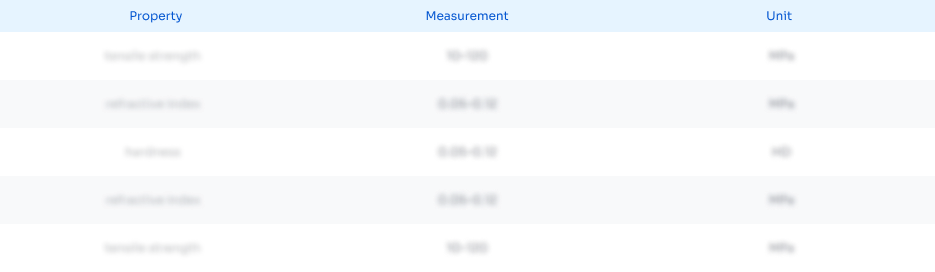
Abstract
Description
Claims
Application Information
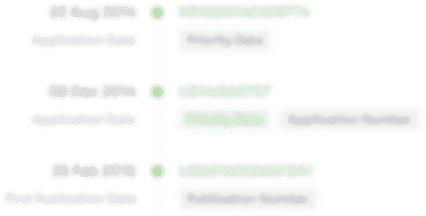
- R&D Engineer
- R&D Manager
- IP Professional
- Industry Leading Data Capabilities
- Powerful AI technology
- Patent DNA Extraction
Browse by: Latest US Patents, China's latest patents, Technical Efficacy Thesaurus, Application Domain, Technology Topic, Popular Technical Reports.
© 2024 PatSnap. All rights reserved.Legal|Privacy policy|Modern Slavery Act Transparency Statement|Sitemap|About US| Contact US: help@patsnap.com