A vibration data fault classification method based on depth domain adaptation
A technology of fault classification and vibration data, applied in the fields of instruments, character and pattern recognition, computer parts, etc., can solve problems such as poor diagnosis effect
- Summary
- Abstract
- Description
- Claims
- Application Information
AI Technical Summary
Problems solved by technology
Method used
Image
Examples
Embodiment Construction
[0066] The following will clearly and completely describe the technical solutions in the embodiments of the present invention with reference to the accompanying drawings in the embodiments of the present invention. Obviously, the described embodiments are only some, not all, embodiments of the present invention. Based on the embodiments of the present invention, all other embodiments obtained by persons of ordinary skill in the art without making creative efforts belong to the protection scope of the present invention.
[0067] Depend on figure 1 As shown, a vibration data fault classification method based on deep domain self-adaptation, first constructs a fault classification model using sample data, and then uses the fault classification model to diagnose and classify the vibration data obtained in real time from the sensor of the target device.
[0068] The present invention constructs a classifier based on the sample data of the source domain, and then combines the domain ...
PUM
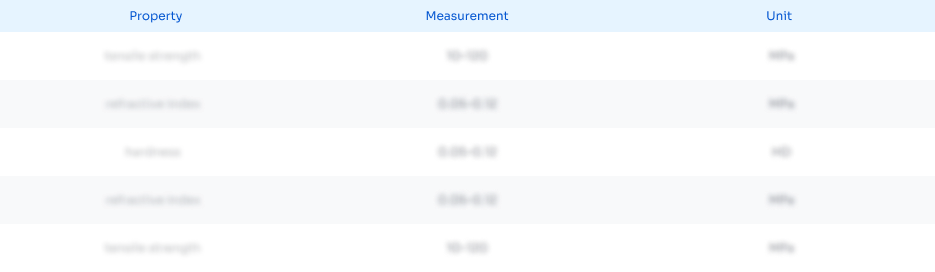
Abstract
Description
Claims
Application Information
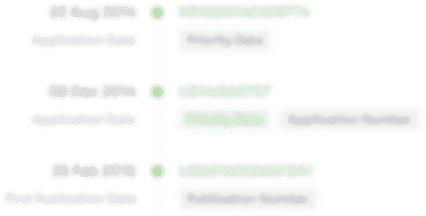
- R&D Engineer
- R&D Manager
- IP Professional
- Industry Leading Data Capabilities
- Powerful AI technology
- Patent DNA Extraction
Browse by: Latest US Patents, China's latest patents, Technical Efficacy Thesaurus, Application Domain, Technology Topic, Popular Technical Reports.
© 2024 PatSnap. All rights reserved.Legal|Privacy policy|Modern Slavery Act Transparency Statement|Sitemap|About US| Contact US: help@patsnap.com