Fault prediction method and device for industrial equipment based on LSTM circulating neural network
A cyclic neural network and fault prediction technology, applied in neural learning methods, biological neural network models, neural architectures, etc., can solve the problems of loss of ability to guide maintenance decisions, underutilization of advantages and characteristics, and reduced prediction accuracy. Achieve the effect of avoiding insufficient prediction accuracy and realizing equipment performance and remaining life
- Summary
- Abstract
- Description
- Claims
- Application Information
AI Technical Summary
Problems solved by technology
Method used
Image
Examples
Embodiment Construction
[0039] Embodiments of the present invention are described in detail below, examples of which are shown in the drawings, wherein the same or similar reference numerals designate the same or similar elements or elements having the same or similar functions throughout. The embodiments described below by referring to the figures are exemplary and are intended to explain the present invention and should not be construed as limiting the present invention.
[0040] The method and device for predicting industrial equipment faults based on LSTM cyclic neural network according to the embodiments of the present invention will be described below with reference to the accompanying drawings.
[0041] figure 1 It is a flowchart of an industrial equipment failure prediction method based on LSTM cyclic neural network according to an embodiment of the present invention.
[0042] like figure 1 As shown, this LSTM cycle neural network-based industrial equipment failure prediction method include...
PUM
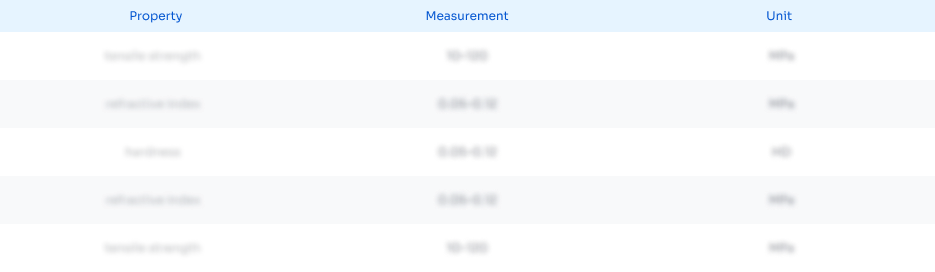
Abstract
Description
Claims
Application Information
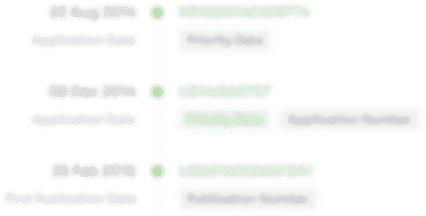
- R&D Engineer
- R&D Manager
- IP Professional
- Industry Leading Data Capabilities
- Powerful AI technology
- Patent DNA Extraction
Browse by: Latest US Patents, China's latest patents, Technical Efficacy Thesaurus, Application Domain, Technology Topic, Popular Technical Reports.
© 2024 PatSnap. All rights reserved.Legal|Privacy policy|Modern Slavery Act Transparency Statement|Sitemap|About US| Contact US: help@patsnap.com