A road traffic flow forecasting method
A flow forecasting and road traffic technology, applied in traffic flow detection, instruments, calculations, etc., can solve problems such as difficulty in finding the global optimal solution or satisfactory solution, premature convergence accuracy of fruit fly algorithm, low generalization ability, etc., to improve The effect of global optimal performance, improved generalization performance, and low generalization prediction error
- Summary
- Abstract
- Description
- Claims
- Application Information
AI Technical Summary
Problems solved by technology
Method used
Image
Examples
Embodiment 1
[0028] Embodiment 1: as Figure 1-3 Shown, a road traffic flow prediction method, the method includes the following steps:
[0029] (1) Establishment of the model: Assuming that the cross-section traffic flow at the intersection to be predicted at time t is v(t), the embedding dimension is m, and the time interval is τ, then there is a set x(i)={v(t-τ) }, v(t-2*τ)... v(t-(m-1)*τ)}, the sample {x(i), v(t)} is a training sample, the task is to find out through the model Features of historical state sequence data to predict traffic flow at intersections in the future;
[0030] (2) Establishment of forecasting model: the main solution of regression analysis is to find out the dependency relationship f(x) for the input x(i), and predict the formula of the least squares support vector machine theory according to the traffic flow, as follows;
[0031]
[0032] where x is the factor affecting the traffic flow, x i is the i-th sample of the input, K(x,x i ) is a kernel function, ...
PUM
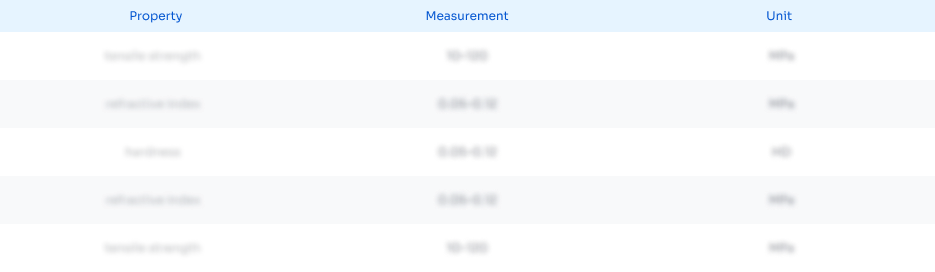
Abstract
Description
Claims
Application Information
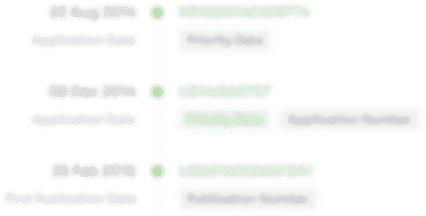
- R&D Engineer
- R&D Manager
- IP Professional
- Industry Leading Data Capabilities
- Powerful AI technology
- Patent DNA Extraction
Browse by: Latest US Patents, China's latest patents, Technical Efficacy Thesaurus, Application Domain, Technology Topic, Popular Technical Reports.
© 2024 PatSnap. All rights reserved.Legal|Privacy policy|Modern Slavery Act Transparency Statement|Sitemap|About US| Contact US: help@patsnap.com