Small sample and zero sample image classification method based on metric learning and meta-learning
A technology of metric learning and classification methods, applied in the field of small-sample and zero-sample image classification based on metric learning and meta-learning, which can solve problems such as different data distribution, classification noise, and inability to apply multiple different data sets
- Summary
- Abstract
- Description
- Claims
- Application Information
AI Technical Summary
Problems solved by technology
Method used
Image
Examples
Embodiment Construction
[0052] The accompanying drawings are for illustrative purposes only and cannot be construed as limiting the patent;
[0053] In order to better illustrate this embodiment, some parts in the drawings will be omitted, enlarged or reduced, and do not represent the size of the actual product;
[0054] For those skilled in the art, it is understandable that some well-known structures and descriptions thereof may be omitted in the drawings.
[0055] The technical solutions of the present invention will be further described below in conjunction with the accompanying drawings and embodiments.
[0056] like figure 1 As shown, it is a method flow chart of the small-sample and zero-sample image classification method based on metric learning and meta-learning in this embodiment.
[0057] A few-shot and zero-shot image classification method based on metric learning and meta-learning, including the following steps:
[0058] Step 1: Collect life scene images, and construct training data s...
PUM
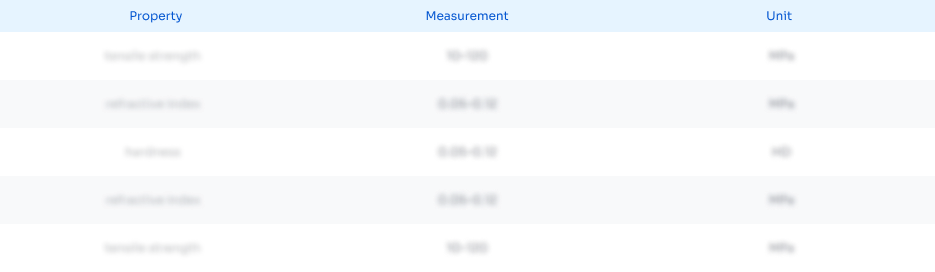
Abstract
Description
Claims
Application Information
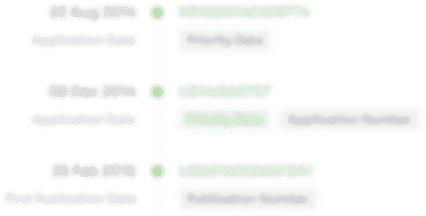
- R&D Engineer
- R&D Manager
- IP Professional
- Industry Leading Data Capabilities
- Powerful AI technology
- Patent DNA Extraction
Browse by: Latest US Patents, China's latest patents, Technical Efficacy Thesaurus, Application Domain, Technology Topic, Popular Technical Reports.
© 2024 PatSnap. All rights reserved.Legal|Privacy policy|Modern Slavery Act Transparency Statement|Sitemap|About US| Contact US: help@patsnap.com