A Graph Learning Model Based on Reconstruction Graph
A technology for learning models and reconstructing graphs, applied in the field of graph learning models based on reconstructed graphs, can solve the problems of affecting image labeling accuracy, label co-occurrence imbalance, low label recall rate, etc., to overcome internal connection problems and labels. The effect of the co-occurrence imbalance problem on
- Summary
- Abstract
- Description
- Claims
- Application Information
AI Technical Summary
Problems solved by technology
Method used
Image
Examples
Embodiment Construction
[0029] The specific implementation manners of the present invention will be further described below in conjunction with the accompanying drawings and technical solutions.
[0030] A graph learning model based on reconstructed graphs, including three stages: graph learning between images, graph learning between labels, and mapping between images and labels.
[0031] The first stage is the image-based graph learning stage. First, for the problem of weak labels in the image dataset, an improved nearest neighbor strategy is designed to increase the labeling probability of weak labels and suppress the labeling probability of high-frequency labels. Then reconstruct the obtained similarity matrix to mine the deep connection between images. This stage includes the selection process of semantic nearest neighbors, the similarity matrix reconstruction process of unlabeled images, and the iterative process of graph learning.
[0032] (1) The selection process of the semantic nearest neig...
PUM
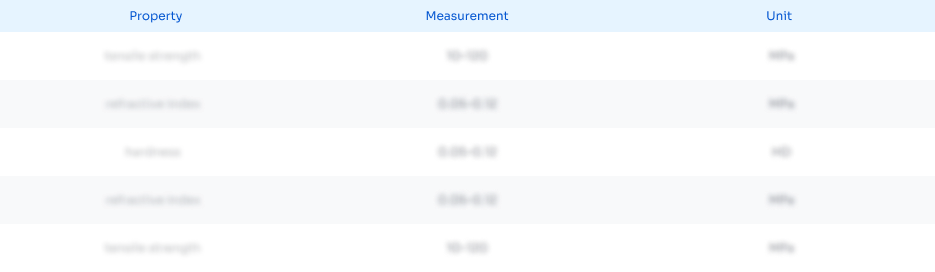
Abstract
Description
Claims
Application Information
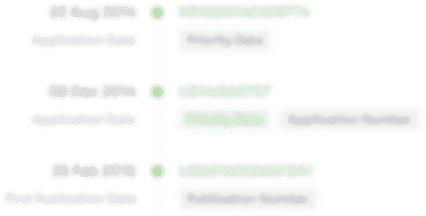
- R&D Engineer
- R&D Manager
- IP Professional
- Industry Leading Data Capabilities
- Powerful AI technology
- Patent DNA Extraction
Browse by: Latest US Patents, China's latest patents, Technical Efficacy Thesaurus, Application Domain, Technology Topic, Popular Technical Reports.
© 2024 PatSnap. All rights reserved.Legal|Privacy policy|Modern Slavery Act Transparency Statement|Sitemap|About US| Contact US: help@patsnap.com