Ballistocardiogram signal atrial fibrillation computer-aided diagnosis method based on transfer learning
A computer-aided, cardiac shock signal technology, applied in computer-aided medical procedures, neural learning methods, diagnosis, etc., can solve problems such as lack of database, weak BCG signal amplitude, inappropriate machine learning methods, etc., to expand the field of daily application Effect
- Summary
- Abstract
- Description
- Claims
- Application Information
AI Technical Summary
Problems solved by technology
Method used
Image
Examples
Embodiment Construction
[0033] The specific implementation manners of the present invention will be further described in detail below in conjunction with the accompanying drawings and embodiments. The following examples are used to illustrate the present invention, but are not intended to limit the scope of the present invention.
[0034] In this embodiment, a computer-aided diagnosis method for cardiac shock signal atrial fibrillation based on transfer learning, such as figure 1 shown, including the following steps:
[0035] Step 1: Preprocess the synchronously measured ECG signal, BCG signal and the ECG signal in the existing atrial fibrillation disease database, and set the frame length of all signal data to 24 seconds according to the characteristics of atrial fibrillation disease, as The input vector of the neural network;
[0036] Step 1.1: Collect synchronous ECG signals and BCG signals of the same subject, and normalize the signals respectively to obtain measured data;
[0037] Step 1.2: O...
PUM
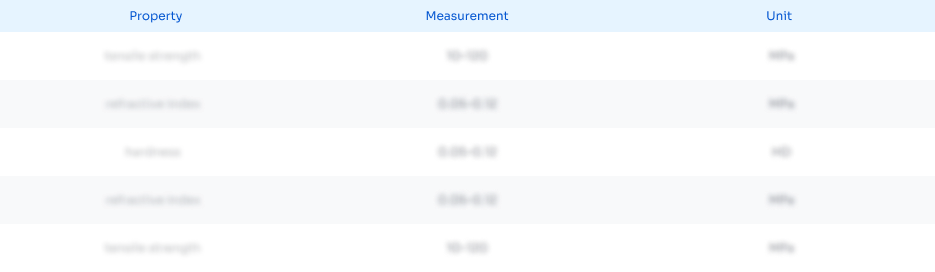
Abstract
Description
Claims
Application Information
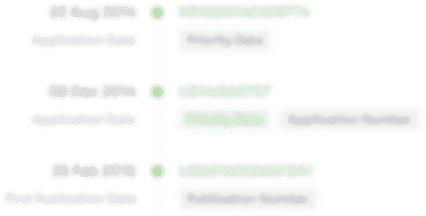
- R&D Engineer
- R&D Manager
- IP Professional
- Industry Leading Data Capabilities
- Powerful AI technology
- Patent DNA Extraction
Browse by: Latest US Patents, China's latest patents, Technical Efficacy Thesaurus, Application Domain, Technology Topic.
© 2024 PatSnap. All rights reserved.Legal|Privacy policy|Modern Slavery Act Transparency Statement|Sitemap