Multi-factor short-term traffic flow prediction method based on neural network LSTM
A technology of neural network and forecasting method, applied in the field of multi-factor short-term traffic flow forecasting based on neural network LSTM, can solve the problems of poor forecasting effect of LSTM forecasting model, achieve accurate and effective forecasting, improve forecasting accuracy, and improve forecasting accuracy Effect
- Summary
- Abstract
- Description
- Claims
- Application Information
AI Technical Summary
Problems solved by technology
Method used
Image
Examples
Embodiment 1
[0079] In this example, the data required for the example are provided by the toll station in Xi’an. Xi’an belongs to the temperate zone semi-humid continental monsoon climate, with four distinct seasons, mild climate and moderate rainfall. Most of the rainy seasons are concentrated in spring and summer, so in the example The data in June 2018 is counted every 15 minutes. Observing the data, from June 16th to June 18th, the light rain turned cloudy for three consecutive days. At this time, the data from June 1st to 18th is used as the training set, and June 19th is used as the test set to predict the traffic flow on June 19th. According to the previous weather data, it is known that June 19th is also cloudy , the weather conditions are different from the previous three days, and the test results are shown in Figure 2. Then delete the data from June 16th to June 18th to exclude the case of three consecutive days of light rain turning cloudy, and then use the data after excludi...
example 2
[0081] In this example, in order to consider the impact of holidays on traffic flow forecasting, the data in February of the Spring Festival in 2018 is selected as the training set, because according to the local climate conditions, winter is cold and less rainy and snowy, which minimizes the impact of weather factors. Because the Spring Festival in February is the most important day of the year, and about 15 days before and after the Spring Festival is the peak period of Spring Festival travel, the selection of data for the Spring Festival in February fully illustrates the impact of holidays on traffic flow. Use the data in February as the training set to train in the LSTM model, and use the data on March 1st as the test set to make predictions. The results are shown in Figure 4(a) and Figure 4(b). The mse and rmse are relatively large. And the R square is less than 0.92. According to the neural network LSTM model, the prediction effect of this model is better, and the R squar...
PUM
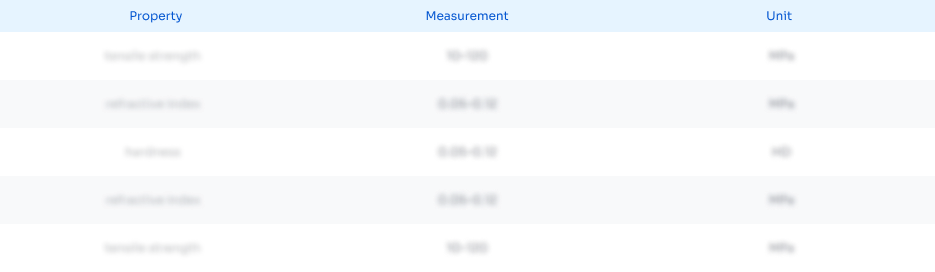
Abstract
Description
Claims
Application Information
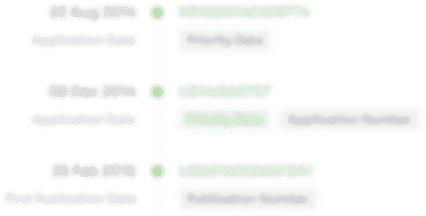
- R&D
- Intellectual Property
- Life Sciences
- Materials
- Tech Scout
- Unparalleled Data Quality
- Higher Quality Content
- 60% Fewer Hallucinations
Browse by: Latest US Patents, China's latest patents, Technical Efficacy Thesaurus, Application Domain, Technology Topic, Popular Technical Reports.
© 2025 PatSnap. All rights reserved.Legal|Privacy policy|Modern Slavery Act Transparency Statement|Sitemap|About US| Contact US: help@patsnap.com