Short-term load prediction method based on C-means clustering fuzzy rough set
A technology of short-term load forecasting and average value clustering, applied in forecasting, character and pattern recognition, instruments, etc., can solve the problems of slow calculation speed and low prediction accuracy, improve power supply reliability, solve slow calculation speed, and improve power grid Planning the effect of safe and efficient operation
- Summary
- Abstract
- Description
- Claims
- Application Information
AI Technical Summary
Problems solved by technology
Method used
Image
Examples
Embodiment 1
[0058] A short-term load forecasting method based on C-means clustering fuzzy rough sets, comprising the following steps:
[0059] S1. Collect historical load data and data of load influencing factors from the energy management system. In this embodiment 1, take a time interval of 15 minutes as a sample unit to collect historical load data for 30 days before the forecast date and loads for N days before the forecast date Influencing factor data, all data are randomly divided into training sets and prediction sets, and load influencing factors are used as condition attributes, and load is used as decision attribute to construct an initial attribute decision table as shown in Table 1; wherein, in this embodiment 1, load Influencing factors include weather, policies, seasons, months, and holidays.
[0060]
[0061]
[0062] Table 1
[0063] S2. According to the condition attribute and the decision attribute, use the fuzzy C-means clustering method to construct the attribut...
PUM
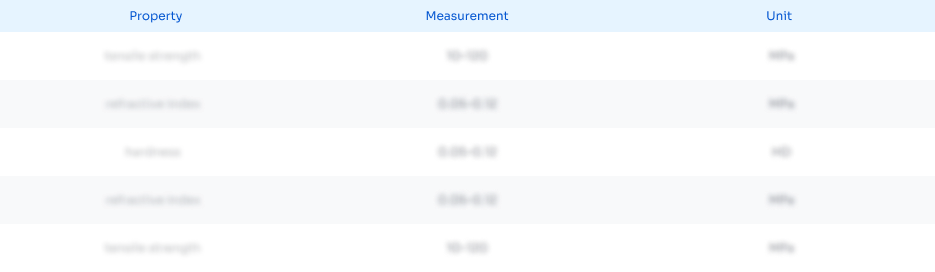
Abstract
Description
Claims
Application Information
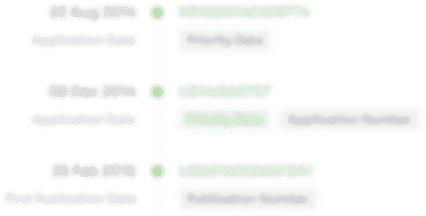
- R&D Engineer
- R&D Manager
- IP Professional
- Industry Leading Data Capabilities
- Powerful AI technology
- Patent DNA Extraction
Browse by: Latest US Patents, China's latest patents, Technical Efficacy Thesaurus, Application Domain, Technology Topic.
© 2024 PatSnap. All rights reserved.Legal|Privacy policy|Modern Slavery Act Transparency Statement|Sitemap