Video classification method based on recurrent neural network
A recurrent neural network and video classification technology, applied in the field of video information mining, can solve the problems of computing resources and time resource consumption, inability to respond to events in real time, large errors, etc., and achieve the effect of reducing video classification errors
- Summary
- Abstract
- Description
- Claims
- Application Information
AI Technical Summary
Problems solved by technology
Method used
Image
Examples
Embodiment Construction
[0024] Specific embodiments of the present invention will be described below in conjunction with the accompanying drawings, so that those skilled in the art can better understand the present invention. It should be noted that in the following description, when detailed descriptions of known functions and designs may dilute the main content of the present invention, these descriptions will be omitted here.
[0025] In the prior art, video classification prediction is mostly based on RNN, CNN or improved methods of these two methods. However, such neural network-based approaches are often considered to lack interpretability. At the same time, the improvement of video classification models (RNN, CNN) is accompanied by a huge number of parameters and an increase in computational complexity. These complex video classification models cannot be effectively deployed on low-cost devices. The present invention innovatively uses Taylor series to explain the gated recurrent unit (a type...
PUM
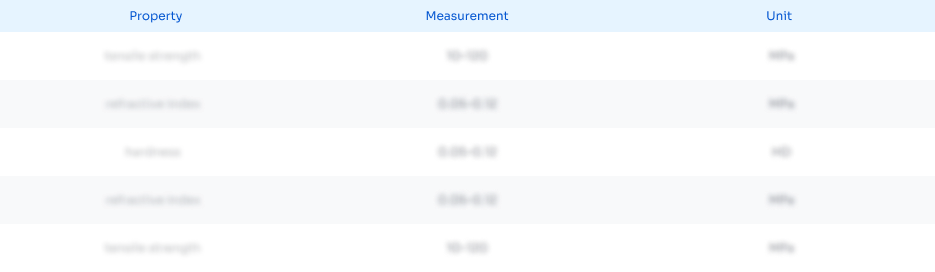
Abstract
Description
Claims
Application Information
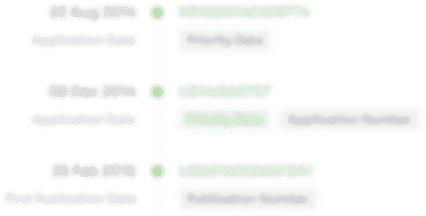
- R&D Engineer
- R&D Manager
- IP Professional
- Industry Leading Data Capabilities
- Powerful AI technology
- Patent DNA Extraction
Browse by: Latest US Patents, China's latest patents, Technical Efficacy Thesaurus, Application Domain, Technology Topic, Popular Technical Reports.
© 2024 PatSnap. All rights reserved.Legal|Privacy policy|Modern Slavery Act Transparency Statement|Sitemap|About US| Contact US: help@patsnap.com