Pixel-level label automatic generation model construction and automatic generation method and device
A technology for automatic generation and construction of methods, applied in biological neural network models, neural architectures, character and pattern recognition, etc., can solve the problems of lack of a large number of training labels, weakly supervised semantic segmentation, etc., to expand the scope of application, improve Semantic segmentation effect, the effect of improving segmentation accuracy
- Summary
- Abstract
- Description
- Claims
- Application Information
AI Technical Summary
Problems solved by technology
Method used
Image
Examples
Embodiment 1
[0075] In this embodiment, a method for constructing a pixel-level automatic label generation model is disclosed, which is used to obtain an automatic label generation model for an image to be labeled.
[0076] Such as figure 1 shown, follow the steps below:
[0077] Step 1. Obtain an existing image set that is semantically similar to the image to be labeled, and obtain a semantically similar image set;
[0078] or
[0079] Obtain multiple single background images containing the object to be labeled in the image to be labeled, and obtain a simple image set;
[0080] Using the semantically similar image set or simple image set to train the deep neural network to obtain a pre-labeled model;
[0081] In this embodiment, one of two methods is used to obtain the pre-marking model. When the existing image set has an image set similar to the object to be marked in the image to be marked, directly use the existing image set to train the deep neural network. , to obtain a pre-annot...
Embodiment 2
[0113] A method for automatically generating pixel-level labels, which is performed in the following steps:
[0114] Step A, obtaining the image to be labeled;
[0115] Step B, using the pixel-level label automatic generation model construction method in Embodiment 1 to construct an automatic label generation model for the image to be labeled;
[0116] Step C. Input the image to be labeled into the automatic label generation model obtained in step B, and output image pixel-level labels.
[0117] In this embodiment, images of different plant species in the "Orchid" family are collected as images to be labeled such as figure 2 shown.
[0118] When using the pixel-level label automatic generation model construction method in Embodiment 1 to construct the label automatic generation model of the image to be marked, the pre-labeled model is obtained using the existing image set PASCAL VOC 2012 data set as a semantically similar image set, PASCAL VOC 2012 The dataset contains 20 ...
Embodiment 3
[0124] In this embodiment, the branch image in the complex background image is obtained as the image to be labeled such as Figure 4 shown.
[0125] When using the pixel-level label automatic generation model construction method in Example 1 to construct the label automatic generation model of the image to be labeled, the pre-labeled model was obtained because the domain "tree branch" semantics was not found on the public dataset with pixel-level labels similar categories, so a simple image set is obtained first by acquiring a single background image, such as Figure 5 As shown, the ResNet101 in DeepLabV3+ is selected as the deep neural network to obtain the pre-labeled model, and then the automatic label generation model is obtained through pre-labeled model training. A batch size of 8 is used for training, and the initial learning rate is set to 0.007, divided by 10 every 5 epochs. Weight decay and momentum are set to 0.0002 and 0.9 respectively.
[0126] Will Figure 4 ...
PUM
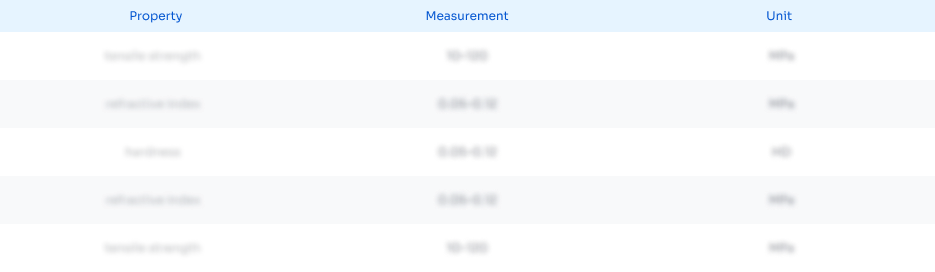
Abstract
Description
Claims
Application Information
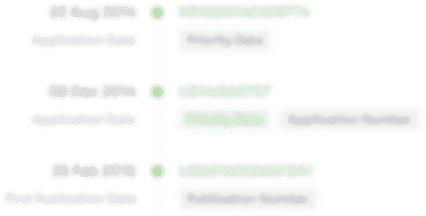
- R&D Engineer
- R&D Manager
- IP Professional
- Industry Leading Data Capabilities
- Powerful AI technology
- Patent DNA Extraction
Browse by: Latest US Patents, China's latest patents, Technical Efficacy Thesaurus, Application Domain, Technology Topic, Popular Technical Reports.
© 2024 PatSnap. All rights reserved.Legal|Privacy policy|Modern Slavery Act Transparency Statement|Sitemap|About US| Contact US: help@patsnap.com