Cross-domain semantic segmentation method based on three-branch cross training
A cross-training and semantic segmentation technology, applied in neural learning methods, image analysis, instruments, etc., can solve problems such as poor cross-domain segmentation results
- Summary
- Abstract
- Description
- Claims
- Application Information
AI Technical Summary
Problems solved by technology
Method used
Image
Examples
Embodiment Construction
[0070] In order to avoid low-precision pseudo-labels affecting the model learning target domain-specific knowledge, the present invention proposes three-branch cross-training. Any two branches generate new and unique target domain knowledge to train the third branch, so that each branch can ensure that it is in the There are unique expression distributions in the same feature space. They share a feature extractor, which ensures that the three branches have feature distributions that can learn from each other in the same feature space. The different structures of the three branches also ensure the diversity of the initial feature distribution. Subsequently, the pseudo-label of each branch is different from the probability distribution of the three branches through a special method of selecting pseudo-labels, which ensures that each branch can obtain new and supplementary target domain knowledge without additional constraints, which further enhances the their diversity. This e...
PUM
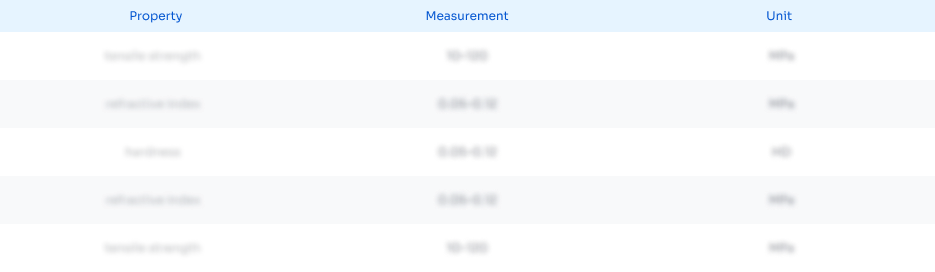
Abstract
Description
Claims
Application Information
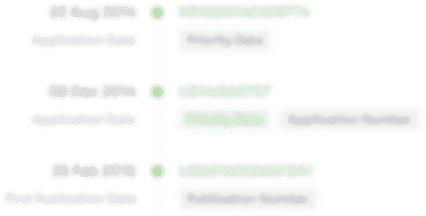
- R&D Engineer
- R&D Manager
- IP Professional
- Industry Leading Data Capabilities
- Powerful AI technology
- Patent DNA Extraction
Browse by: Latest US Patents, China's latest patents, Technical Efficacy Thesaurus, Application Domain, Technology Topic, Popular Technical Reports.
© 2024 PatSnap. All rights reserved.Legal|Privacy policy|Modern Slavery Act Transparency Statement|Sitemap|About US| Contact US: help@patsnap.com