Graph theory correlation theory-based anomaly detection method
An anomaly detection and theoretical technology, applied in the field of anomaly detection, can solve problems such as the redundancy of anomaly detection process, reduce the accuracy of anomaly detection methods, and the loss of abnormal points, so as to reduce time complexity and space complexity, and reduce useless data Set, improve the effect of robustness
- Summary
- Abstract
- Description
- Claims
- Application Information
AI Technical Summary
Problems solved by technology
Method used
Image
Examples
Embodiment Construction
[0061] The technical solutions of the present invention will be clearly and completely described below in conjunction with embodiments. Obviously, the described embodiments are only a part of the embodiments of the present invention, rather than all the embodiments. Based on the embodiments of the present invention, all other embodiments obtained by those of ordinary skill in the art without creative work shall fall within the protection scope of the present invention.
[0062] The present invention is to solve the problem of abnormal point detection under the condition of large data volume and high dimension; when abnormal detection is performed on a large data set, the abnormal detection process on the whole data set will produce a large amount of abnormal detection process redundancy , Which greatly consumes computing resources. In the process of judging abnormal points, only using an abnormal basis as the judgment criterion will lead to the appearance of some normal data point...
PUM
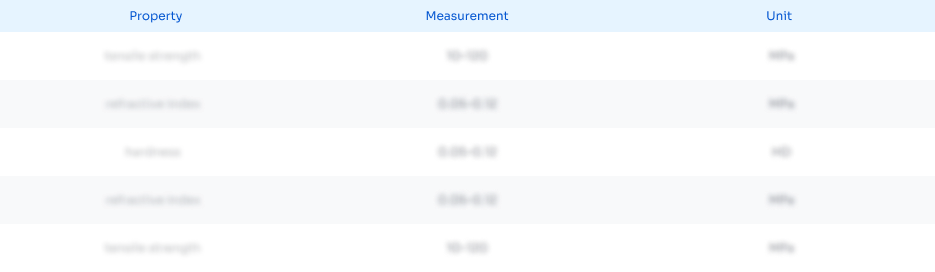
Abstract
Description
Claims
Application Information
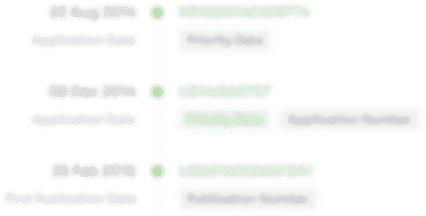
- R&D
- Intellectual Property
- Life Sciences
- Materials
- Tech Scout
- Unparalleled Data Quality
- Higher Quality Content
- 60% Fewer Hallucinations
Browse by: Latest US Patents, China's latest patents, Technical Efficacy Thesaurus, Application Domain, Technology Topic, Popular Technical Reports.
© 2025 PatSnap. All rights reserved.Legal|Privacy policy|Modern Slavery Act Transparency Statement|Sitemap|About US| Contact US: help@patsnap.com