Multi-granularity answer sorting multi-document machine reading understanding method
A reading comprehension, multi-document technology, applied in the field of machine reading comprehension, can solve problems such as poor model representation and generalization ability, inability to integrate multi-granularity question and answer correlation, and limited model input length.
- Summary
- Abstract
- Description
- Claims
- Application Information
AI Technical Summary
Problems solved by technology
Method used
Image
Examples
Embodiment 1
[0073] figure 1 A multi-document machine reading comprehension method for multi-granularity answer sorting according to the present invention and a flow chart of this embodiment;
[0074] from figure 1 It can be seen that the present invention comprises the following steps:
[0075] Step A: question and document joint feature representation;
[0076] Specifically, obtain questions and multiple documents and perform document splitting, input sequence vectorization, and text semantic representation;
[0077] Specifically in this embodiment, this step A corresponds to steps 1 to 5 in the summary of the invention;
[0078] Obtain the question and multiple documents and split the documents, specifically: split the documents into sequence lengths that can be input by the model according to the predefined sliding window length and sliding distance, specifically corresponding to steps 1 to 2 in the content of the invention;
[0079] Input sequence vectorization, that is, to obtain...
Embodiment 2
[0091] This example will start with the question "Is a gecko a beneficial insect?" Document 1 "Gecko is a beneficial insect. It eats mosquitoes, flies and insects. It looks ugly but is actually a beneficial insect and does not bite people." Document 2 "First of all, it is harmless to humans. You should not You will be afraid when you see it in the house, but it will not bite, and it also eats mosquitoes and bugs. Geckos have no harmful characteristics, and a few species may be poisonous." Document 3 "Geckos are reptiles that belong to Lizards have a lot of medicinal value, beneficial insects, nocturnal, and like to hunt flies in places with lights at night, they are not harmful to people, and they are national second-class protected animals.” An example is described in the present invention. The specific operation steps of a multi-document machine reading comprehension method for multi-granularity answer sorting are described in detail.
[0092] The processing flow of a multi-...
Embodiment 3
[0111] In order to further verify the effectiveness of a multi-document machine reading comprehension method for multi-granularity answer sorting in the present invention, this embodiment uses the 270,000 question-multi-document corpus used in Example 2, and the question-multi-document corpus comes from Baidu Company 2017 DuReader, a large-scale Chinese multi-document machine reading comprehension dataset released in 2016, each question corresponds to 5 documents from Baidu search engine or Baidu Knowing Community. The dataset has a document answer label for each question, and the 5 documents are searched The order in which the engine actually returns.
PUM
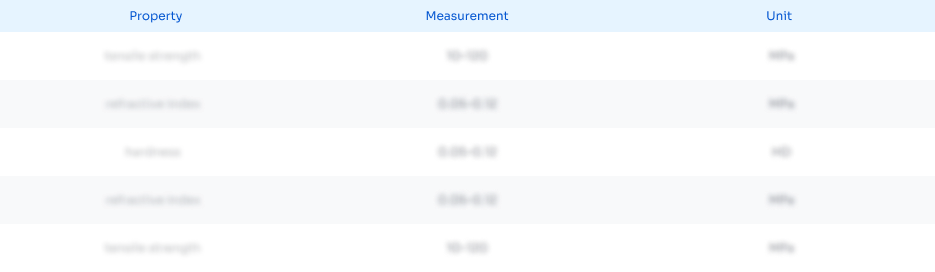
Abstract
Description
Claims
Application Information
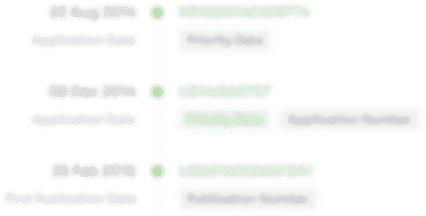
- R&D Engineer
- R&D Manager
- IP Professional
- Industry Leading Data Capabilities
- Powerful AI technology
- Patent DNA Extraction
Browse by: Latest US Patents, China's latest patents, Technical Efficacy Thesaurus, Application Domain, Technology Topic, Popular Technical Reports.
© 2024 PatSnap. All rights reserved.Legal|Privacy policy|Modern Slavery Act Transparency Statement|Sitemap|About US| Contact US: help@patsnap.com