A Dimensionality Reduction Sparse STAP Method and Device Based on Uncertain Prior Knowledge
A priori knowledge and dimensionality reduction technology, applied in measuring devices, radio wave reflection/re-radiation, instruments, etc., can solve problems such as high complexity, uncertain prior knowledge, and poor implementation of airborne radar clutter suppression , to achieve the effect of improving realizability and reducing computational complexity
- Summary
- Abstract
- Description
- Claims
- Application Information
AI Technical Summary
Problems solved by technology
Method used
Image
Examples
no. 1 example
[0033] In order to solve the unextensive adaptive processing algorithm in the related art, the implementation of poor realization in the inhibition of the airborne radar clutter, this embodiment proposes an uncertain prior knowledge. Designated STAP method, such as figure 1 The basic flow diagram of the drop-dimensional STAP method provided in this embodiment is shown, and the desired STAP method proposed in this embodiment includes the following steps:
[0034] Step 101, using the discrete Fourier transform to the radar reception signal to the radar, resulting in a hetero-whallar fortune reduction dimension measurement model.
[0035] In an alternative embodiment of this embodiment, see if figure 2 The flow schematic of the first-order degradation method, the implementation of step 101, specifically includes the steps of:
[0036] Step 201. Based on the reduction conversion matrix, the radar receive signal is reduced to the radar reception signal, and the interference component i...
no. 2 example
[0098] In order to more description of the contents of the present invention, the present embodiment illustrates the effects of different parameters to the present invention and the present invention in the sample data, SINR performance sparse recovery by simulation data.
[0099] In the simulation, the radar parameters are set as follows: Airborne Radar V p = 125m / s, h p = 4000m; D 0= 0.0625m and PRF = 4000Hz. Assuming clutter given distance into unit 361, a noise obeys each independent zero-mean complex Gaussian noise with variance satisfy a zero mean complex Gaussian distribution. The present embodiment is obtained by averaging the results of the simulation independent Monte Carlo experiment 500 times.
[0100] In the present embodiment, it is assumed coprime array has N = 6 sensors, which is coprime to N 1 = 2, N 2 = 3, a number of pulses of coherent processing interval (CPI) is M = 18, heteroaryl noise ratio CNR = 40dB, M e = 15, the number of training samples each experime...
no. 3 example
[0103] In order to solve high complexity adaptive processing algorithms air employed in the related art, the realizability in airborne radar clutter suppression poor technical problem, the present embodiment shows an embodiment based on a priori knowledge uncertainty dimensionality reduction sparse STAP device details, see Figure 11 , STAP sparse dimension reduction apparatus according to the present embodiment includes:
[0104] Order a dimension reduction module 1101, using the discrete Fourier transform for the received radar signal to a dimensionality reduction step to obtain a first-order noise reduction dimensional sparse measurement model;
[0105] Second order dimension reduction module 1102, for second-order and first-order dimension reduction to reduce clutter dimensional sparse measurement uncertainty model parameters a priori knowledge of the radar-dimensional matrix structure by reducing second order dimensionality reduction matrix of second order, resulting in a hybr...
PUM
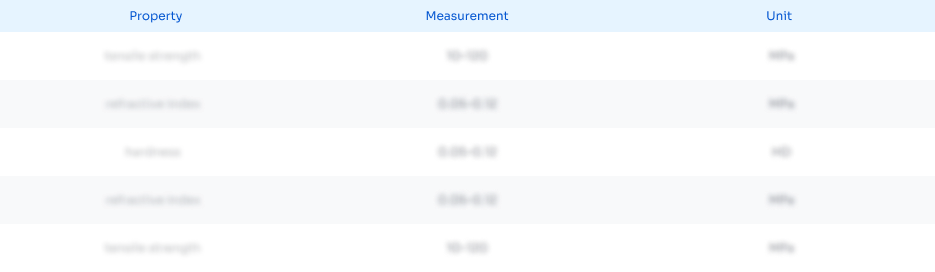
Abstract
Description
Claims
Application Information
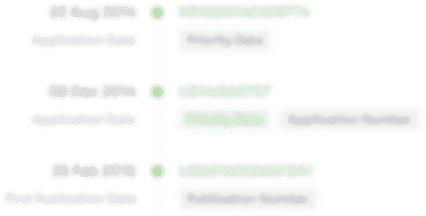
- R&D Engineer
- R&D Manager
- IP Professional
- Industry Leading Data Capabilities
- Powerful AI technology
- Patent DNA Extraction
Browse by: Latest US Patents, China's latest patents, Technical Efficacy Thesaurus, Application Domain, Technology Topic, Popular Technical Reports.
© 2024 PatSnap. All rights reserved.Legal|Privacy policy|Modern Slavery Act Transparency Statement|Sitemap|About US| Contact US: help@patsnap.com