Multi-modal brain glioma image segmentation method of adaptive attention gate
A glioma, multimodal technology, applied in image analysis, image enhancement, image data processing and other directions, can solve the problems of high heterogeneity of glioma images and blurred partition boundaries, and achieve the effect of improving segmentation performance
- Summary
- Abstract
- Description
- Claims
- Application Information
AI Technical Summary
Problems solved by technology
Method used
Image
Examples
Embodiment Construction
[0027] In order to make the purpose, technical solutions and advantages of the embodiments of the present invention clearer, the technical solutions in the embodiments of the present invention will be clearly and completely described below in conjunction with the drawings in the embodiments of the present invention. Obviously, the described embodiments It is only some embodiments of the present invention, but not all embodiments. Based on the embodiments of the present invention, all other embodiments obtained by persons of ordinary skill in the art without making creative efforts belong to the protection scope of the present invention.
[0028] Such as Figure 1-Figure 3 In combination with the above, the present invention discloses a method for multi-modal brain glioma image segmentation with an adaptive attention gate with multi-level features. The method includes the following steps:
[0029] S1: Preprocessing the MRI glioma image data to obtain a data sample set;
[003...
PUM
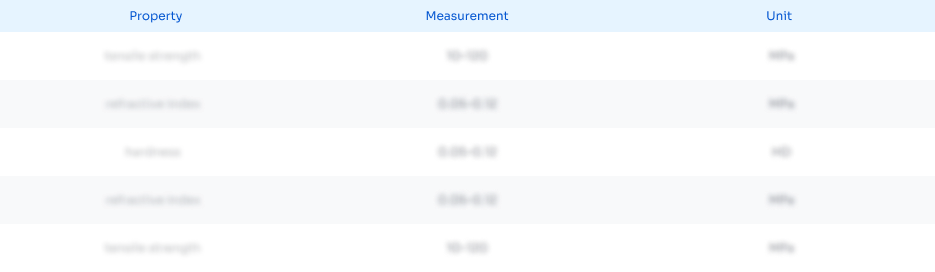
Abstract
Description
Claims
Application Information
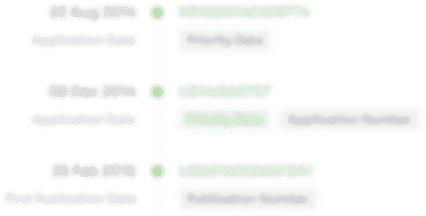
- R&D
- Intellectual Property
- Life Sciences
- Materials
- Tech Scout
- Unparalleled Data Quality
- Higher Quality Content
- 60% Fewer Hallucinations
Browse by: Latest US Patents, China's latest patents, Technical Efficacy Thesaurus, Application Domain, Technology Topic, Popular Technical Reports.
© 2025 PatSnap. All rights reserved.Legal|Privacy policy|Modern Slavery Act Transparency Statement|Sitemap|About US| Contact US: help@patsnap.com