Ear CT (Computed Tomography) image vestibular segmentation method for mixing 2D (Two Dimensional) and 3D (Three Dimensional) convolutional neural networks
A convolutional neural network and CT image technology, applied in ear CT image diagnosis, computer image processing, and deep learning fields, can solve problems such as difficult training, lack of categories, and huge demand for computing resources, so as to improve work efficiency and quality, The effect of high segmentation accuracy and excellent segmentation performance
- Summary
- Abstract
- Description
- Claims
- Application Information
AI Technical Summary
Problems solved by technology
Method used
Image
Examples
Embodiment Construction
[0024] The present invention will be described in detail below in conjunction with the accompanying drawings and embodiments.
[0025] A hybrid 2D and 3D convolutional neural network vestibular segmentation method for ear CT images, the implementation process of the method is as follows:
[0026] Step 1: Build the dataset
[0027] The data set used in the present invention is divided into three parts, including a training set of 82 cases with a total of 570 CT images, a verification set of 10 cases with a total of 72 CT images and a test set of 10 cases with a total of 72 CT images. The data is annotated by radiologists with rich clinical experience, and all CT images are annotated at the voxel level of the vestibule.
[0028] In the present invention, only axial images are used. Therefore, multi-planar reconstruction and standardization operations are performed on the spiral CT scan images of the temporal bone of the ear to keep the imaging parameters consistent within a ce...
PUM
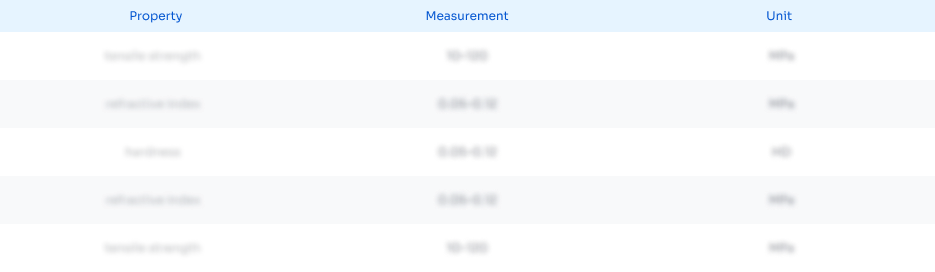
Abstract
Description
Claims
Application Information
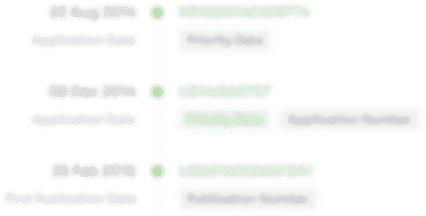
- R&D
- Intellectual Property
- Life Sciences
- Materials
- Tech Scout
- Unparalleled Data Quality
- Higher Quality Content
- 60% Fewer Hallucinations
Browse by: Latest US Patents, China's latest patents, Technical Efficacy Thesaurus, Application Domain, Technology Topic, Popular Technical Reports.
© 2025 PatSnap. All rights reserved.Legal|Privacy policy|Modern Slavery Act Transparency Statement|Sitemap|About US| Contact US: help@patsnap.com