Medical image segmentation method, device and equipment and readable storage medium
A medical image and image segmentation technology, applied in the field of medical image processing, can solve the problems of high requirement of medical image detail, loss of medical image segmentation, affecting quantitative calculation of cardiac ejection volume, etc.
- Summary
- Abstract
- Description
- Claims
- Application Information
AI Technical Summary
Problems solved by technology
Method used
Image
Examples
Embodiment 1
[0065] In order to facilitate those skilled in the art to better understand the medical image segmentation method provided by the embodiment of the present invention, the medical image segmentation method provided by the embodiment of the present invention will be compared and described below in conjunction with the prior art.
[0066] The input data of medical image segmentation is generally CT data or nuclear magnetic data, and a case usually contains multiple CT images or nuclear magnetic images. There are many deep learning image segmentation methods, no matter which method is used, it is necessary to design a loss function and optimize the training of the network.
[0067] In the prior art, it is assumed that the input image dimension of a case is 512×512, and there are n images in the case. For the segmentation network, the calculation of the loss function of the network model usually adopts the method of cross entropy loss, and the calculation method is as follows:
[0068] T...
Embodiment 2
[0114] In order to increase the segmentation accuracy of the central region of organs and tissues. On the basis of the first embodiment, the embodiment of the present invention can also weight the loss (loss value) of the central area to increase the proportion of the loss value of the central area.
[0115] That is, in the medical image segmentation method provided by the embodiment of the present invention, the training process of the deep learning image segmentation network may also include:
[0116] Determine the area corresponding to the label matrix in the medical image training sample as the central area of the organization;
[0117] Correspondingly, when the loss function is used to calculate the loss value, the loss weight is added to the pixels corresponding to the edge enhancement region in the training segmentation result, including:
[0118] When using the loss function to calculate the loss value, increase the loss weight for the corresponding pixels in the edge enhanc...
Embodiment 3
[0130] In order to flexibly train the deep learning image segmentation network, it can also calculate the loss value corresponding to the edge enhancement area, the loss value corresponding to the center area of the organization, the loss value of all areas, and weight the different area loss values according to different weight ratios Calculate to obtain the overall loss value.
[0131] Please refer to Figure 4 , The overall loss value calculation process, including:
[0132] S21: Calculate the edge enhancement loss value corresponding to the edge enhancement area by using the loss weight corresponding to each pixel in the edge enhancement area;
[0133] S22: Calculate the center loss value corresponding to the center area of the organization by using the loss weight of each pixel in the center area of the organization;
[0134] S23. Calculate ordinary loss values corresponding to all regions of the medical image training sample;
[0135] S24. Perform weighted superposition...
PUM
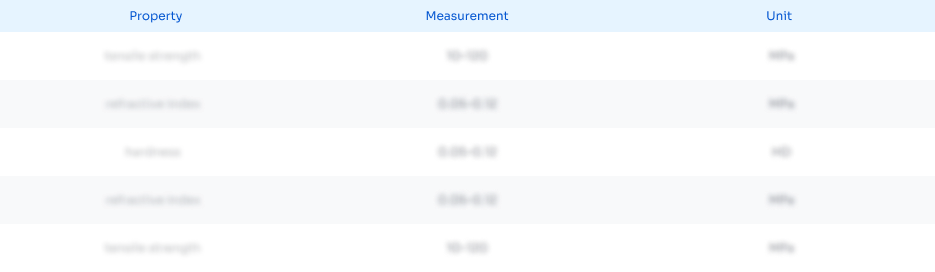
Abstract
Description
Claims
Application Information
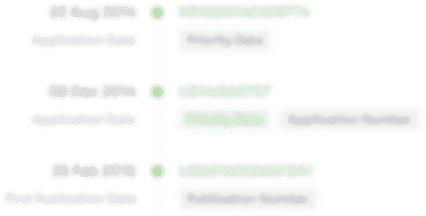
- R&D
- Intellectual Property
- Life Sciences
- Materials
- Tech Scout
- Unparalleled Data Quality
- Higher Quality Content
- 60% Fewer Hallucinations
Browse by: Latest US Patents, China's latest patents, Technical Efficacy Thesaurus, Application Domain, Technology Topic, Popular Technical Reports.
© 2025 PatSnap. All rights reserved.Legal|Privacy policy|Modern Slavery Act Transparency Statement|Sitemap|About US| Contact US: help@patsnap.com