Memory normalization network layer method based on double forward propagation algorithm
A forward propagation and normalization technology, applied in the design of the network layer and the research field of neural network training algorithms, can solve the problems of feature distribution deviation, inconsistency, model deviation, etc., to improve training stability and reduce training instability. problems, the effect of reducing the problem of inaccurate calculation of statistics
- Summary
- Abstract
- Description
- Claims
- Application Information
AI Technical Summary
Problems solved by technology
Method used
Image
Examples
Embodiment 1
[0042] A kind of memory normalization network layer method based on the double forward propagation algorithm of the present embodiment 1, such as figure 1 shown, including the following steps:
[0043] S1. Estimate the statistical information of multiple batches in the memory storage and normalize the training data;
[0044] S2. After performing the standard forward and backward propagation, the double forward propagation algorithm performs an additional forward propagation to reduce the distribution deviation of the network middle layer information caused by the update of the neural network model.
[0045] The memory is stored as a real-valued vector with dimension K, which records the statistics of the data distribution recorded in the latest K iterations.
[0046] Such as figure 2 As shown, the distribution of the training samples is estimated according to the statistical information of multiple batches in the memory storage, and the specific calculation method is as fo...
PUM
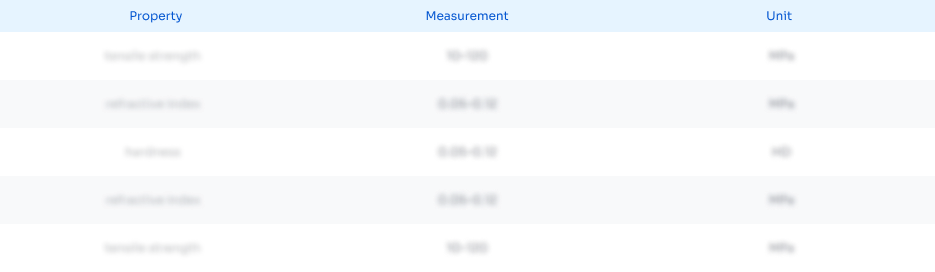
Abstract
Description
Claims
Application Information
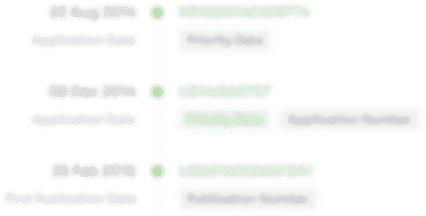
- R&D
- Intellectual Property
- Life Sciences
- Materials
- Tech Scout
- Unparalleled Data Quality
- Higher Quality Content
- 60% Fewer Hallucinations
Browse by: Latest US Patents, China's latest patents, Technical Efficacy Thesaurus, Application Domain, Technology Topic, Popular Technical Reports.
© 2025 PatSnap. All rights reserved.Legal|Privacy policy|Modern Slavery Act Transparency Statement|Sitemap|About US| Contact US: help@patsnap.com