Small sample multi-classification method based on nested integrated depth support vector machine
A support vector machine, multi-classification technology, applied in neural learning methods, computer parts, character and pattern recognition, etc., can solve problems such as high computational cost, deviation of sample distribution estimates, large amounts of data, etc., to improve classification accuracy , in exchange for the flexible effect of the kernel function
- Summary
- Abstract
- Description
- Claims
- Application Information
AI Technical Summary
Problems solved by technology
Method used
Image
Examples
Embodiment 1
[0066] A small-sample multi-classification method based on nested ensemble deep support vector machine, such as figure 1 The structural diagram of the nested ensemble DSVM algorithm is shown. It is a deep support vector machine (Deep Support Vector Machine, DSVM) algorithm with three different single-kernel functions as a sub-classifier to build a base classifier; it will use a "one-to-one" strategy to convert the multi-category classification problem into multiple binary Classification problems; through the AdaBoost framework to complete multi-classification tasks, including:
[0067] (1) Sub-classifier model
[0068] figure 1 The principle of two-layer DSVM is described, and the input with label training data for ,
[0069] ; where, are called training sample points; these training samples, after containing in a DSVM core layer To extract the corresponding features, given to ;Finally, by a main SVM named M, the above eigenvalues are processed and predicted...
Embodiment 2
[0112] We choose a separate SVM and endow it with the same kernel functions as the subclassifier DSVM, namely radial basis kernel function, Sigmod kernel function and polynomial kernel function; the same training data and test data are used to select the SVM The kernel function is trained and tested to obtain the correlation coefficient and hyperplane equation expression that needs to be obtained; calculate the geometric mean distance from all test samples to the hyperplane according to the obtained parameter values; use all the correct test samples to obtain The reciprocal of the mean square error of the distance between the distance and the geometric mean distance of all test samples is used as the weight value corresponding to the kernel function, and this is given to the base classifiers DSVM1, DSVM2, and DSVM3; Table 1:
[0113]
[0114] From Table 1, it can be seen that each sub-classifier The weight value after selecting the kernel function . Among them, the radi...
Embodiment 3
[0116] We built a system for detecting human objects through walls. The experimental equipment used in this system is a P410MRM single base station radar module with small size, low power consumption and simple operation. It is equipped with antenna ports for dual antenna operation. In experiments, the system uses this module to detect human targets behind walls and obtain reflected human status signals. Meanwhile, three indoor experimental schemes were designed. Including the state of no one behind the wall, the state of one person breathing rapidly behind the wall, and the state of two people walking slowly at 0.1m / s behind the wall. The wall involved in the experiment is a brick wall. The thickness of the brick wall is 25 cm. The distance between the human target and the wall is 100 cm. The distance between the radar equipment and the wall is 60 cm, and the human target is separated from the brick wall. On the basis of the above-mentioned experimental system, we colle...
PUM
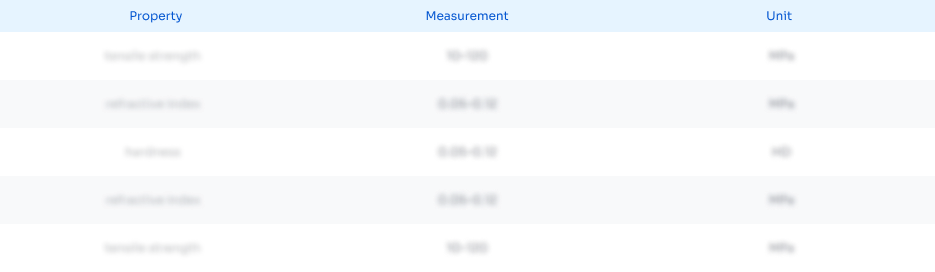
Abstract
Description
Claims
Application Information
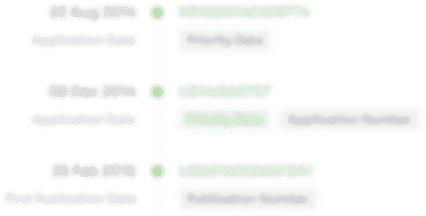
- R&D
- Intellectual Property
- Life Sciences
- Materials
- Tech Scout
- Unparalleled Data Quality
- Higher Quality Content
- 60% Fewer Hallucinations
Browse by: Latest US Patents, China's latest patents, Technical Efficacy Thesaurus, Application Domain, Technology Topic, Popular Technical Reports.
© 2025 PatSnap. All rights reserved.Legal|Privacy policy|Modern Slavery Act Transparency Statement|Sitemap|About US| Contact US: help@patsnap.com