A Domain Adaptive Privacy Protection Method Based on Differential Privacy for Deep Neural Networks
A deep neural network and differential privacy technology, applied in the field of artificial intelligence security, can solve problems such as a lot of time and effort, and the difficulty of classifying models, achieving the effect of low actual loss, strong practicability, and protection of personal privacy
- Summary
- Abstract
- Description
- Claims
- Application Information
AI Technical Summary
Problems solved by technology
Method used
Image
Examples
Embodiment Construction
[0037] As shown in the figure, the deep neural network-oriented domain adaptation privacy protection method based on differential privacy designed by the present invention includes the following steps:
[0038] 1) as figure 1 As shown, a deep feed-forward neural network model with two processes is defined for a server with source domain images and a user with target domain images. In process 1, the model is trained to predict labels for source-domain images, where the source-domain image labels are known. In process 2, the model is trained to predict the labels of the source and target domain images, where the source domain image label is defined as 1 and the target domain image label is defined as 0.
[0039] 2) as figure 1As shown, this model can be decomposed into three parts, the feature extraction part, the label prediction part and the domain classification part. The data in both stages is mapped in the feature extraction part. This model uses the Alexnet structure t...
PUM
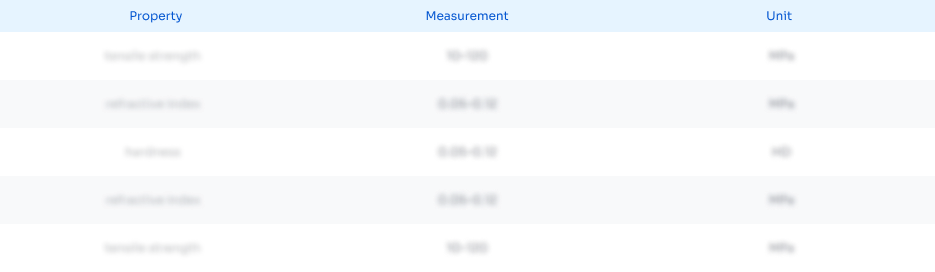
Abstract
Description
Claims
Application Information
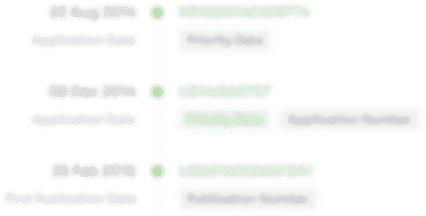
- R&D
- Intellectual Property
- Life Sciences
- Materials
- Tech Scout
- Unparalleled Data Quality
- Higher Quality Content
- 60% Fewer Hallucinations
Browse by: Latest US Patents, China's latest patents, Technical Efficacy Thesaurus, Application Domain, Technology Topic, Popular Technical Reports.
© 2025 PatSnap. All rights reserved.Legal|Privacy policy|Modern Slavery Act Transparency Statement|Sitemap|About US| Contact US: help@patsnap.com