Unsupervised multi-view feature selection method based on graph learning and view weight learning
A feature selection method and feature selection technology, applied in character and pattern recognition, instruments, computer components, etc., can solve the problems of not being able to obtain the optimal similarity graph, assigning view weights without parameters, etc.
- Summary
- Abstract
- Description
- Claims
- Application Information
AI Technical Summary
Problems solved by technology
Method used
Image
Examples
Embodiment Construction
[0056] The present invention will be further described below in conjunction with the accompanying drawings and embodiments, and the present invention includes but not limited to the following embodiments.
[0057] Such as figure 1 As shown, the present invention provides an unsupervised multi-view feature selection method based on graph learning and view weight learning, and its basic implementation process is as follows:
[0058] 1. Data preprocessing
[0059] After inputting the original multi-view data X, first of all for each view data X (v) Do preprocessing:
[0060] (1) Calculate the mean value of each row, and subtract each element from the mean value of the row to centralize the data;
[0061] (2) Calculate the 2-norm value of each row of data after centering, and then divide each element value at this time by the 2-norm value of the row where it is located to complete the data preprocessing process.
[0062] Use the preprocessed data as the data to be used in subs...
PUM
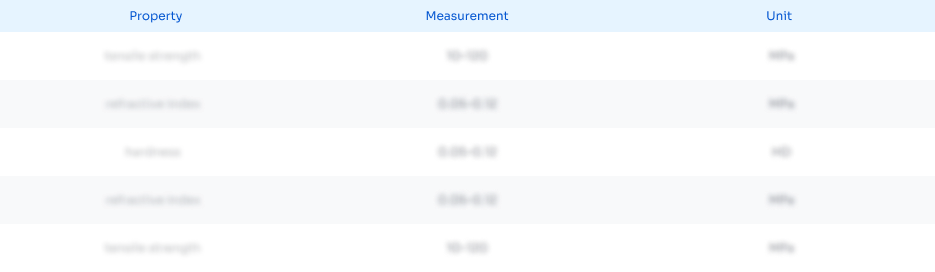
Abstract
Description
Claims
Application Information
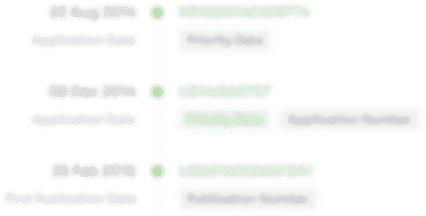
- R&D Engineer
- R&D Manager
- IP Professional
- Industry Leading Data Capabilities
- Powerful AI technology
- Patent DNA Extraction
Browse by: Latest US Patents, China's latest patents, Technical Efficacy Thesaurus, Application Domain, Technology Topic, Popular Technical Reports.
© 2024 PatSnap. All rights reserved.Legal|Privacy policy|Modern Slavery Act Transparency Statement|Sitemap|About US| Contact US: help@patsnap.com