Video target real-time tracking method and system based on depth feature fusion and adaptive correlation filtering
A depth feature and correlation filtering technology, applied in the field of target tracking, can solve problems such as insufficient ability of multi-layer depth feature to represent the target, target moving out of the field of view and target rotation, feature redundancy, etc., to achieve strong target background discrimination ability, strong target The effect of improving expression ability and representation ability
- Summary
- Abstract
- Description
- Claims
- Application Information
AI Technical Summary
Problems solved by technology
Method used
Image
Examples
Embodiment Construction
[0074] The applicant believes that extracting features from the deep neural network model is the most time-consuming step, and the most direct speed-up method is to use a lightweight deep neural network model. However, the multi-layer deep features extracted from the lightweight model have insufficient ability to represent the target, limited ability to distinguish the background and the target, and there is redundancy between features, resulting in additional calculations. At the same time, target tracking tasks often face specific problems such as target deformation, target occlusion, target moving out of view, and target rotation. Using existing correlation filtering algorithms is prone to tracker drift or even tracking failure.
[0075] For this reason, the present invention proposes a multi-layer deep feature fusion strategy based on canonical correlation analysis (CCA) based on deep feature fusion and adaptive correlation filtering to improve feature expression ability an...
PUM
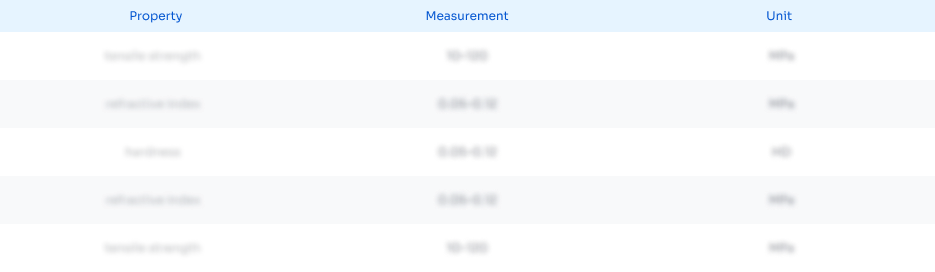
Abstract
Description
Claims
Application Information
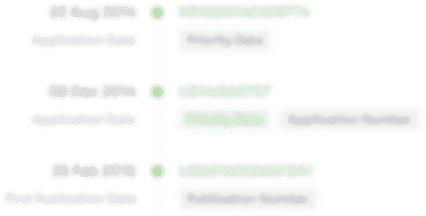
- R&D Engineer
- R&D Manager
- IP Professional
- Industry Leading Data Capabilities
- Powerful AI technology
- Patent DNA Extraction
Browse by: Latest US Patents, China's latest patents, Technical Efficacy Thesaurus, Application Domain, Technology Topic.
© 2024 PatSnap. All rights reserved.Legal|Privacy policy|Modern Slavery Act Transparency Statement|Sitemap