Width learning system based on multi-feature extraction
A learning system and multi-feature technology, applied in the field of wide learning system, can solve the problems of less classification accuracy and no model training time
- Summary
- Abstract
- Description
- Claims
- Application Information
AI Technical Summary
Problems solved by technology
Method used
Image
Examples
Embodiment Construction
[0065] The present invention will be further described below in conjunction with the accompanying drawings and embodiments.
[0066] The width learning system based on multi-feature extraction in this embodiment includes four sub-width learning systems, and each sub-width learning system includes a feature node, an enhancement node, and a sub-node.
[0067] Each of the sub-width learning systems first extracts an image feature from the image dataset, and the image features extracted by each sub-width learning system are different from each other. The first sub-width learning system extracts the HOG feature of the image data set, and the second sub-width learning system extracts the HOG feature of the image data set. The width learning system extracts the color features of the image dataset, the third sub-width learning system extracts the K-means features of the image dataset, and the fourth sub-width learning system extracts the convolution features of the image dataset; each ...
PUM
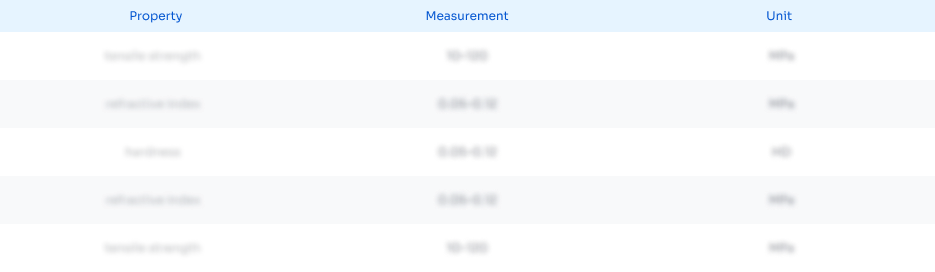
Abstract
Description
Claims
Application Information
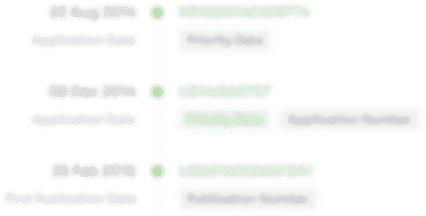
- R&D Engineer
- R&D Manager
- IP Professional
- Industry Leading Data Capabilities
- Powerful AI technology
- Patent DNA Extraction
Browse by: Latest US Patents, China's latest patents, Technical Efficacy Thesaurus, Application Domain, Technology Topic, Popular Technical Reports.
© 2024 PatSnap. All rights reserved.Legal|Privacy policy|Modern Slavery Act Transparency Statement|Sitemap|About US| Contact US: help@patsnap.com