CT image lung lobe recognition method based on neural network
A CT imaging and neural network technology, applied in the field of computer-aided medicine, can solve problems such as errors, achieve high accuracy, high feature learning ability, and increase the complexity of the model
- Summary
- Abstract
- Description
- Claims
- Application Information
AI Technical Summary
Problems solved by technology
Method used
Examples
Embodiment Construction
[0032] The present invention will be further described below.
[0033] A neural network-based CT image lung lobe recognition method, comprising the steps of:
[0034] a) Collect chest CT image data, and label the chest CT data according to background, left upper lobe, left lower lobe, right upper lobe, right middle lobe, and right lower lobe
[0035] A well-converged lung lobe recognition model is obtained through a convolutional neural network, and the specific methods are shown in step b)-step r).
[0036] b) Input the chest CT image X into the residual module C 1 , using the computer through the residual module C 1 The feature output map C is obtained after compound operation processing 1 (X)
[0037] c) Use the computer to output the features in Figure C 1 (X) Perform maximum pooling operation, compress feature map C1 (X), get the updated feature output map C' 1 (X). By compressing the feature map C 1 (X) allows the network to learn the characteristics of position ...
PUM
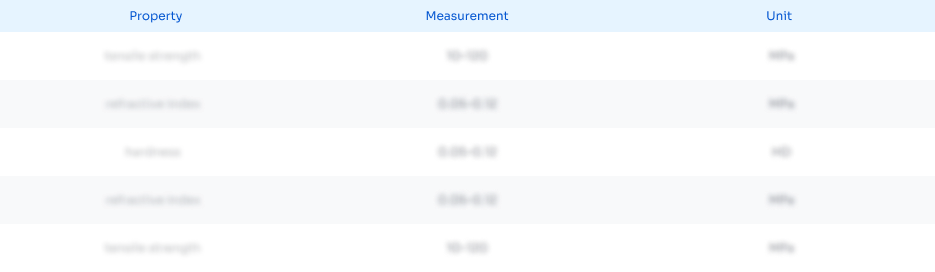
Abstract
Description
Claims
Application Information
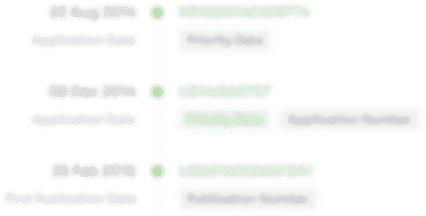
- R&D Engineer
- R&D Manager
- IP Professional
- Industry Leading Data Capabilities
- Powerful AI technology
- Patent DNA Extraction
Browse by: Latest US Patents, China's latest patents, Technical Efficacy Thesaurus, Application Domain, Technology Topic.
© 2024 PatSnap. All rights reserved.Legal|Privacy policy|Modern Slavery Act Transparency Statement|Sitemap