An Essential Image Analysis Method Based on Multi-Scale Attention and Label Loss
An attention, multi-scale technology, applied in the field of image processing, it can solve the problems that the network cannot achieve the separation effect, the reflection map and the light map are not completely separated, etc., to improve the reflection image decomposition quality, generate clear details, and local texture consistency. good effect
- Summary
- Abstract
- Description
- Claims
- Application Information
AI Technical Summary
Problems solved by technology
Method used
Image
Examples
Embodiment
[0170] Such as figure 1 As shown, the multi-scale attention MSA-Net network structure in the present invention is constructed based on the idea of generative confrontation, and is divided into two main components: a generator and a discriminator.
[0171] Such as figure 2 As shown, the generator part consists of an attention subnetwork and a codec subnetwork. The attention sub-network is built based on LSTM components, and the attention map is gradually refined in a 3-level LSTM cascade. Compared with the traditional LSTM structure, the convolutional LSTM structure adds a convolution operation before each activation function inside the traditional LSTM, making LSTM more suitable for the processing of two-dimensional image data. Since the multi-scale information of the image can well reflect the characteristics of the image in different frequency bands, the attention sub-network of the present invention is constructed based on the multi-scale information of the image, and ...
PUM
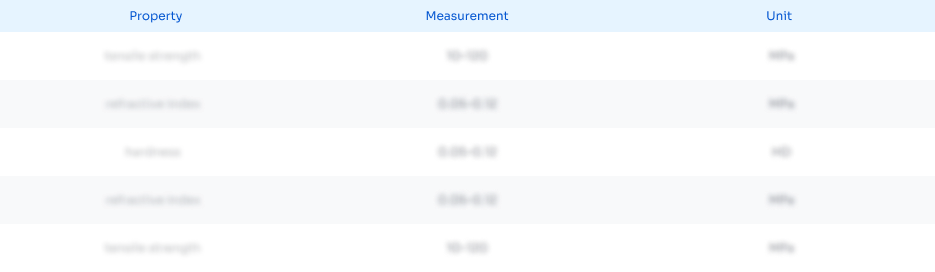
Abstract
Description
Claims
Application Information
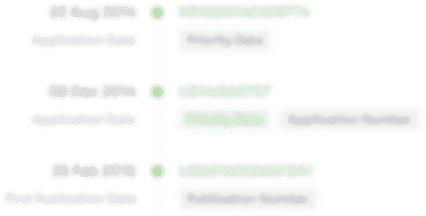
- R&D
- Intellectual Property
- Life Sciences
- Materials
- Tech Scout
- Unparalleled Data Quality
- Higher Quality Content
- 60% Fewer Hallucinations
Browse by: Latest US Patents, China's latest patents, Technical Efficacy Thesaurus, Application Domain, Technology Topic, Popular Technical Reports.
© 2025 PatSnap. All rights reserved.Legal|Privacy policy|Modern Slavery Act Transparency Statement|Sitemap|About US| Contact US: help@patsnap.com