Weak supervision semantic segmentation method and application thereof
A technology of semantic segmentation and weak supervision, applied in the field of computer vision, can solve the problem of low precision of semantic segmentation, achieve the effect of simplifying computational complexity, enhancing expansion efficiency, and improving expansion efficiency
- Summary
- Abstract
- Description
- Claims
- Application Information
AI Technical Summary
Problems solved by technology
Method used
Image
Examples
Embodiment 1
[0040] A weakly supervised semantic segmentation method 100, such as figure 1 As shown, using the pre-trained semantic erasing region expansion classification network for weakly supervised semantic segmentation, the following steps are performed:
[0041]Step 110, performing the first-stage feature extraction and high-level semantic integration classification on the image to be semantically segmented in order to obtain the first category response map corresponding to the image;
[0042] Step 120, erasing the areas with high responsiveness in the first category response map, and performing the second-stage high-level semantic integration classification on the erased category response map to obtain the second category response map;
[0043] Step 130: Add and fuse the corresponding positions of the first category response map and the second category response map respectively to obtain the fused category response map, and perform background threshold cutting processing on the fuse...
Embodiment 2
[0083] An application of any weakly supervised semantic segmentation method as described in Embodiment 1, which is used to perform semantic segmentation on multiple pictures to be semantically segmented to obtain a category segmentation area map, based on multiple pictures to be semantically segmented and their corresponding Class Segmentation Region Maps, Training Semantic Segmentation Networks.
[0084] Another semantic segmentation method uses the above-mentioned semantic segmentation network to perform semantic segmentation on the image to be semantically segmented to complete the semantic segmentation.
[0085] This embodiment adopts the weakly supervised semantic segmentation method described in Embodiment 1. Due to its erasing-type region expansion algorithm based on high-level semantic information, a fused category response map is obtained through multi-stage expansion, and the fused category response map is processed. Region segmentation, the obtained category segment...
Embodiment 3
[0087] A storage medium, in which instructions are stored, and when the computer reads the instructions, the computer is made to execute any weakly supervised semantic segmentation method as described in the first embodiment above and / or as described in the second embodiment above A semantic segmentation method described above.
[0088] The relevant technical solutions are the same as those in Embodiment 1 and Embodiment 2, and will not be repeated here.
PUM
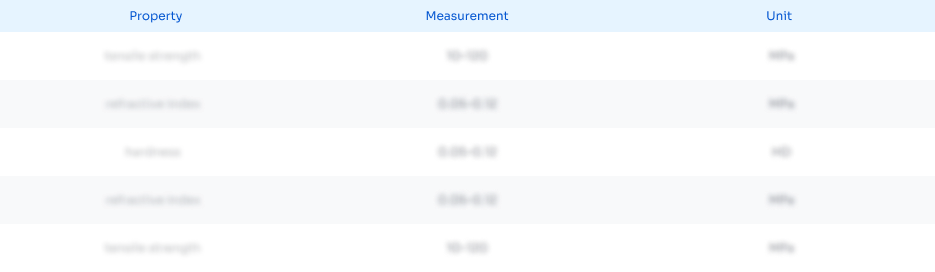
Abstract
Description
Claims
Application Information
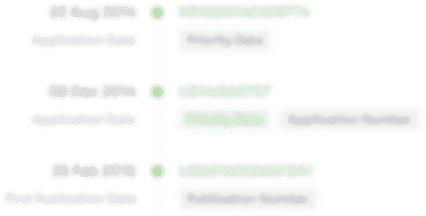
- R&D
- Intellectual Property
- Life Sciences
- Materials
- Tech Scout
- Unparalleled Data Quality
- Higher Quality Content
- 60% Fewer Hallucinations
Browse by: Latest US Patents, China's latest patents, Technical Efficacy Thesaurus, Application Domain, Technology Topic, Popular Technical Reports.
© 2025 PatSnap. All rights reserved.Legal|Privacy policy|Modern Slavery Act Transparency Statement|Sitemap|About US| Contact US: help@patsnap.com