Junk image fine-grained classification method based on incremental learning
An incremental learning, garbage technology, applied in neural learning methods, instruments, biological neural network models, etc., can solve problems such as poor classification accuracy, and achieve the effect of training rapidity guarantee
- Summary
- Abstract
- Description
- Claims
- Application Information
AI Technical Summary
Problems solved by technology
Method used
Image
Examples
Embodiment Construction
[0026]In order to make the technical solution of the present invention clearer, the present invention will be further elaborated below in conjunction with the accompanying drawings. The present invention is concretely realized according to the following steps:
[0027] The first step is to prepare the dataset.
[0028] (1) Prepare image data and label data.
[0029] Constructing a garbage image database of new and old categories: using the Huawei Cloud garbage classification dataset, which contains 19,459 images in 43 categories. The 43-category data set is divided into two categories, old and new, and 30 of them are randomly selected as the old-category data set to train the feature extraction network, and the remaining 13 categories are used as the new-category data set for incremental learning effect testing.
[0030] (2) Preprocess the image.
[0031] Preprocess the image dataset and calculate the dataset mean and standard deviation for normalization. The 30-class old ...
PUM
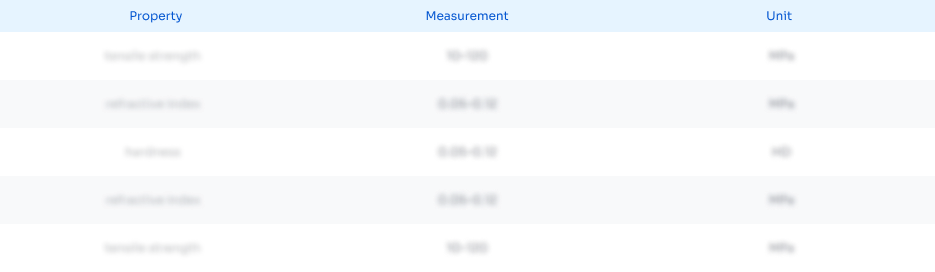
Abstract
Description
Claims
Application Information
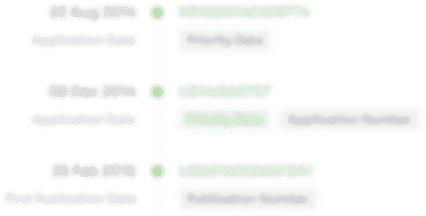
- R&D
- Intellectual Property
- Life Sciences
- Materials
- Tech Scout
- Unparalleled Data Quality
- Higher Quality Content
- 60% Fewer Hallucinations
Browse by: Latest US Patents, China's latest patents, Technical Efficacy Thesaurus, Application Domain, Technology Topic, Popular Technical Reports.
© 2025 PatSnap. All rights reserved.Legal|Privacy policy|Modern Slavery Act Transparency Statement|Sitemap|About US| Contact US: help@patsnap.com