Evolutionary learning method for intelligent monitoring of cutter states
A technology of intelligent monitoring and learning methods, applied in manufacturing tools, complex mathematical operations, measuring/indicating equipment, etc., can solve the problems that the tool state monitoring model cannot adapt to the degradation process of CNC machine tools, so as to improve reliability, avoid failure, The effect of reducing complexity
- Summary
- Abstract
- Description
- Claims
- Application Information
AI Technical Summary
Benefits of technology
Problems solved by technology
Method used
Image
Examples
Embodiment Construction
[0038] In order to make the technical solution and beneficial effects of the present invention clearer, the present invention will be described in detail below in combination with specific implementations of deep-hole boring tool state monitoring and with reference to the accompanying drawings. This embodiment is carried out on the premise of the technical solution of the present invention, and provides detailed implementation and specific operation process, but the protection scope of the present invention is not limited to the following embodiments.
[0039]Taking the processing of deep-hole parts by a certain type of domestic horizontal deep-hole boring machine as an example, the implementation of the present invention will be described in detail.
[0040] The first step, dynamic signal acquisition during deep hole boring
[0041] Vibration signals and noise signals during deep hole boring are collected by piezoelectric three-way acceleration sensors and microphones respect...
PUM
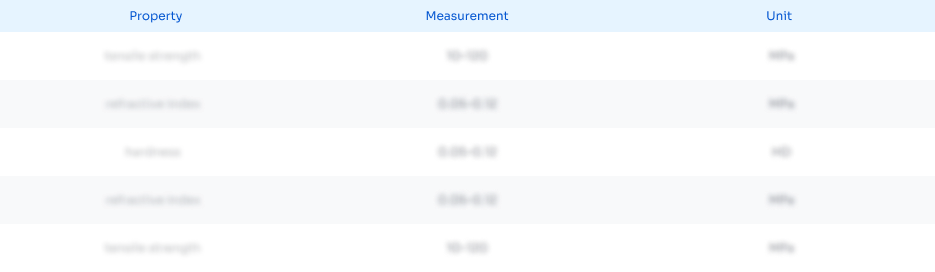
Abstract
Description
Claims
Application Information
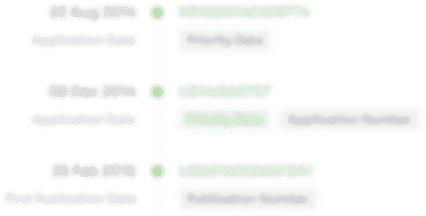
- R&D
- Intellectual Property
- Life Sciences
- Materials
- Tech Scout
- Unparalleled Data Quality
- Higher Quality Content
- 60% Fewer Hallucinations
Browse by: Latest US Patents, China's latest patents, Technical Efficacy Thesaurus, Application Domain, Technology Topic, Popular Technical Reports.
© 2025 PatSnap. All rights reserved.Legal|Privacy policy|Modern Slavery Act Transparency Statement|Sitemap|About US| Contact US: help@patsnap.com