Super-realistic drawing image style migration method based on deep learning
A realism, deep learning technology, applied in image enhancement, image analysis, image data processing and other directions, can solve problems such as affecting the quality of primitives, achieve good image quality, and avoid image generation failures.
- Summary
- Abstract
- Description
- Claims
- Application Information
AI Technical Summary
Problems solved by technology
Method used
Image
Examples
Embodiment 1
[0115] In order to verify the performance of the present invention, this embodiment uses MS-COCO14 as the content map training set, generates a style map data set according to step 1, and iterates 200k times in the RTX2080Ti environment. In order to illustrate the advantages of the present invention, this embodiment uses the same data set under the same conditions to compare the traditional conditional generation network, the existing surrealism (mosaic) style migration method FAMOS (with reference) and the method of the present invention As can be seen from the comparison figure, the method of the present invention has a better performance in terms of surreal style transfer. Specific steps are as follows:
[0116] i. Generating style data sets according to step 1. In this embodiment, samples of two types of style map data are generated, such as Figure 14 with Figure 15 shown;
[0117] ii. Take α=0.4 and β=0.6 in the formula (6), and carry out training with a learning rat...
Embodiment 2
[0121] The method of the present invention can also be used for conventional style transfer tasks, and the specific implementation steps are as follows:
[0122] i. Prepare several images of the same style, and test four styles in the experiment, such as Figure 19 Shown:
[0123] a. A data set composed of several satellite images of Sydney obtained through Google Maps (the resolution of a single image is 1600*800);
[0124] b. Several paintings by Yayoi Kusama;
[0125] c. A Chinese landscape painting;
[0126] d. A green landscape painting style landscape painting;
[0127] ii. For styles (a), (c) and (d), this embodiment uses the (256, 256) slices of the original image; for Yayoi Kusama's painting (c), this embodiment only hopes to learn his strokes , and do not want to learn the content of the original painting, so it is necessary to properly enlarge the original image first, and then cut (256,256) slices from the enlarged image. The slices constitute the style map da...
Embodiment 3
[0132] This embodiment provides a computer-implemented system for migrating surrealist painting image styles based on deep learning, the system includes a processor and a memory, the memory stores a computer program, the processor is a GPU, and calls the computer program Steps 1 to 3 of the deep learning-based surrealist painting image style transfer method described in Example 1.
PUM
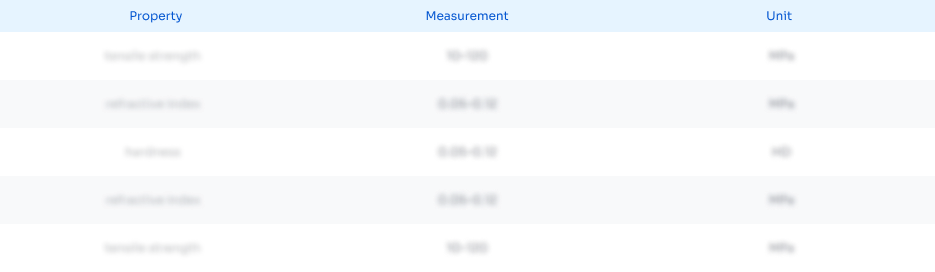
Abstract
Description
Claims
Application Information
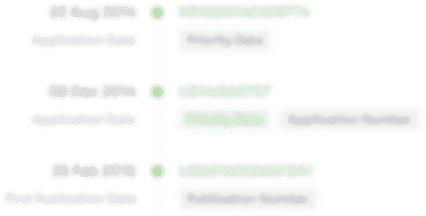
- R&D Engineer
- R&D Manager
- IP Professional
- Industry Leading Data Capabilities
- Powerful AI technology
- Patent DNA Extraction
Browse by: Latest US Patents, China's latest patents, Technical Efficacy Thesaurus, Application Domain, Technology Topic, Popular Technical Reports.
© 2024 PatSnap. All rights reserved.Legal|Privacy policy|Modern Slavery Act Transparency Statement|Sitemap|About US| Contact US: help@patsnap.com