Land utilization classification method for time series remote sensing images
A technology of time series and classification methods, applied in the field of remote sensing image analysis, can solve problems such as hindering the development of classification models and classification effects, hindering the collection of time series remote sensing images, and lack of label samples
- Summary
- Abstract
- Description
- Claims
- Application Information
AI Technical Summary
Problems solved by technology
Method used
Image
Examples
Embodiment Construction
[0034] The present invention will be further described below in conjunction with the embodiments and accompanying drawings, but the present invention is not limited in any way. Any transformation or replacement based on the teaching of the present invention belongs to the protection scope of the present invention.
[0035] Such as figure 1 As shown, a land use classification method for time series remote sensing images, including the following steps:
[0036] Step 1, perform principal component analysis on the multispectral images that make up the time series remote sensing data, and obtain images of three principal components;
[0037] Step 2, pre-train each three-band image and extract the feature image;
[0038] Step 3, the feature images are sequentially input into the semi-supervised convolutional long-term short-term memory network model in chronological order for training;
[0039] Step 4, use the trained model to predict and classify the images in the last phase, and...
PUM
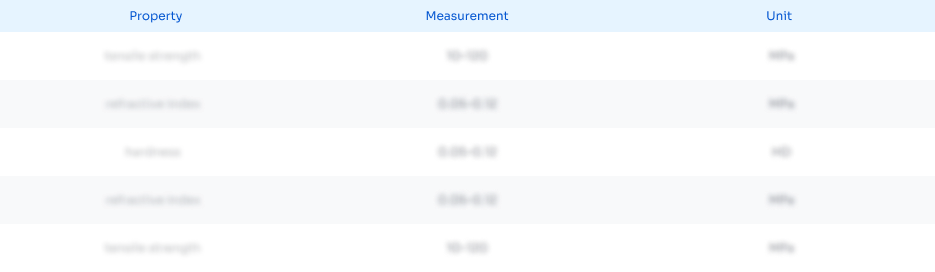
Abstract
Description
Claims
Application Information
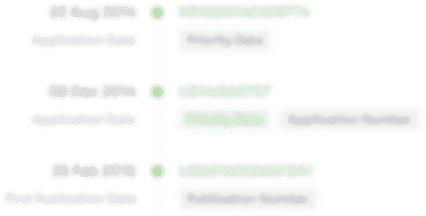
- R&D Engineer
- R&D Manager
- IP Professional
- Industry Leading Data Capabilities
- Powerful AI technology
- Patent DNA Extraction
Browse by: Latest US Patents, China's latest patents, Technical Efficacy Thesaurus, Application Domain, Technology Topic.
© 2024 PatSnap. All rights reserved.Legal|Privacy policy|Modern Slavery Act Transparency Statement|Sitemap