Coarse-grained label-guided deep learning method for cloud region detection in pixel-level remote sensing images
A remote sensing image and deep learning technology, applied in the field of remote sensing and artificial intelligence, can solve the problems of time-consuming, labor-intensive, and labor-intensive labeling work, and achieve the effect of reducing the cost of labeling, improving quality, and improving resolution.
- Summary
- Abstract
- Description
- Claims
- Application Information
AI Technical Summary
Problems solved by technology
Method used
Image
Examples
Embodiment Construction
[0052] In order to facilitate those of ordinary skill in the art to understand and implement the present invention, the present invention will be described in further detail below in conjunction with the accompanying drawings and embodiments. It should be understood that the implementation examples described here are only used to illustrate and explain the present invention, and are not intended to limit this invention.
[0053] A remote sensing image scene classification method based on fault-tolerant deep learning provided by the present invention comprises the following steps:
[0054] Step 1: Input the remote sensing image dataset D={(b n ,y n )|n=1, 2,..., N}, where b n Indicates the nth remote sensing image block in the dataset D; y n Indicates the coarse-grained remote sensing image block-level label corresponding to the nth remote sensing image block, y n has two forms, y n =[1,0] represents the nth remote sensing image block b in the data set D n The label is co...
PUM
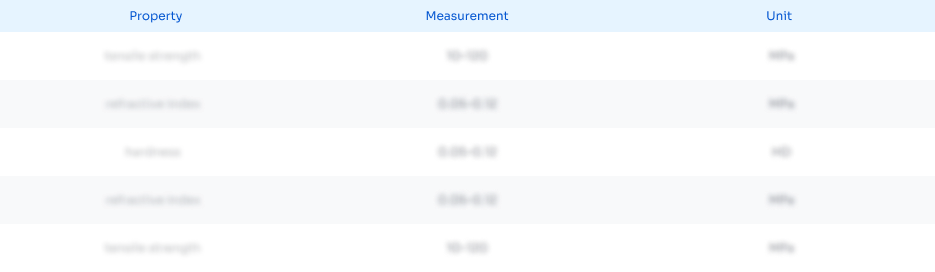
Abstract
Description
Claims
Application Information
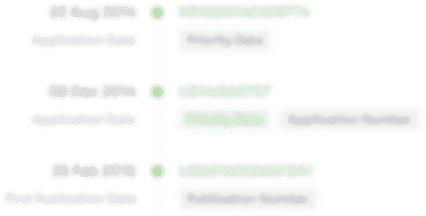
- R&D Engineer
- R&D Manager
- IP Professional
- Industry Leading Data Capabilities
- Powerful AI technology
- Patent DNA Extraction
Browse by: Latest US Patents, China's latest patents, Technical Efficacy Thesaurus, Application Domain, Technology Topic, Popular Technical Reports.
© 2024 PatSnap. All rights reserved.Legal|Privacy policy|Modern Slavery Act Transparency Statement|Sitemap|About US| Contact US: help@patsnap.com