Small sample learning algorithm based on covariance measurement
A learning algorithm and covariance technology, applied in the field of computer vision, can solve problems such as difficult to fully express the complex distribution of categories
- Summary
- Abstract
- Description
- Claims
- Application Information
AI Technical Summary
Problems solved by technology
Method used
Image
Examples
Embodiment Construction
[0040] In order to demonstrate the purpose, features and advantages of the present invention in more detail, the present invention will be further described in detail below in conjunction with the accompanying drawings and specific cases.
[0041] The present invention aims at learning and understanding new concepts (classes) from one example or several examples. Since there are only one or a few labeled samples in each concept, directly adopting machine learning models (such as support vector machine SVM, convolutional neural network CNNs) can easily lead to model overfitting. At this time, the data of each category is too small to fully express a category through these few samples, how to effectively use the auxiliary data set to help learning, and how to learn transferable knowledge from the auxiliary data set is crucial. important. Mainly face three problems that need to be solved in the present invention:
[0042] (1) How to make full use of auxiliary datasets to learn ...
PUM
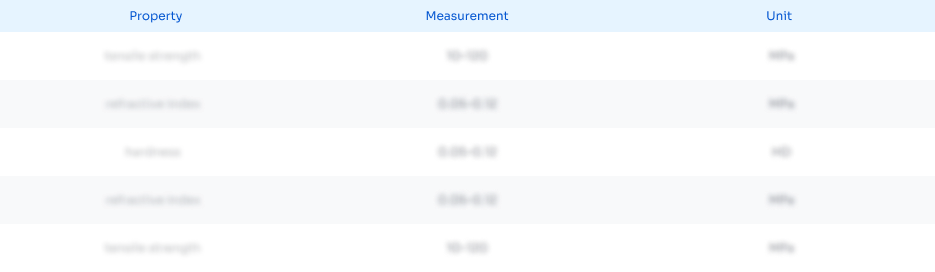
Abstract
Description
Claims
Application Information
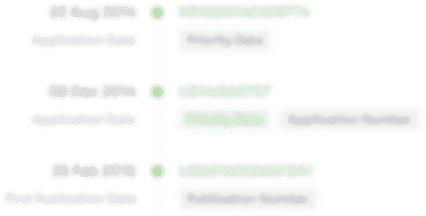
- R&D Engineer
- R&D Manager
- IP Professional
- Industry Leading Data Capabilities
- Powerful AI technology
- Patent DNA Extraction
Browse by: Latest US Patents, China's latest patents, Technical Efficacy Thesaurus, Application Domain, Technology Topic, Popular Technical Reports.
© 2024 PatSnap. All rights reserved.Legal|Privacy policy|Modern Slavery Act Transparency Statement|Sitemap|About US| Contact US: help@patsnap.com