A multi-UAV task decision-making method based on maddpg
A multi-UAV and decision-making method technology, applied in the field of flight control, can solve the problems of increased environmental complexity, unstable environment, and increased variance, and achieve the effect of increasing the level of intelligence, improving combat capabilities, and ensuring survivability
- Summary
- Abstract
- Description
- Claims
- Application Information
AI Technical Summary
Problems solved by technology
Method used
Image
Examples
Embodiment
[0149] In this example, the final network structure is designed as: Actor network structure is fully connected neural network [56; 56; 2], Critic network structure is fully connected neural network [118; 78; 36; 1], two neural networks The hidden layers all use the RELU function as the activation function, such as Image 6 shown. During training, the mini-batch size is 1024, the maximum learning step size (maxepisode) is 30000, the update rate of the auxiliary network is τ=0.01, the learning rate of the critical network is 0.01, and the learning rate of the actor network is 0.001. The AdamOptimizer optimizer is used for learning. The size of the experience pool is 1,000,000. Once the data in the experience pool exceeds the maximum value, the original experience data will be lost, and the constructed multi-UAV task decision-making network will achieve optimal performance.
[0150] The invention initializes the positions of three unmanned aerial vehicles in a designated area in...
PUM
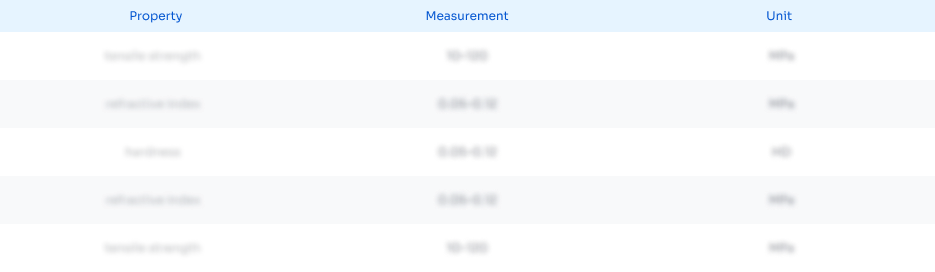
Abstract
Description
Claims
Application Information
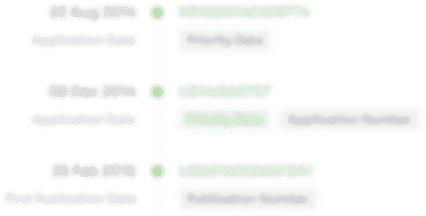
- R&D
- Intellectual Property
- Life Sciences
- Materials
- Tech Scout
- Unparalleled Data Quality
- Higher Quality Content
- 60% Fewer Hallucinations
Browse by: Latest US Patents, China's latest patents, Technical Efficacy Thesaurus, Application Domain, Technology Topic, Popular Technical Reports.
© 2025 PatSnap. All rights reserved.Legal|Privacy policy|Modern Slavery Act Transparency Statement|Sitemap|About US| Contact US: help@patsnap.com