Similarity image retrieval method and system based on sparse coding
An image retrieval and sparse coding technology, applied in the field of image recognition, can solve the problems of relying on training samples, feature extraction methods are not very effective, and there is no targeted measurement standard, so as to achieve the effect of improving learning efficiency
- Summary
- Abstract
- Description
- Claims
- Application Information
AI Technical Summary
Problems solved by technology
Method used
Image
Examples
Embodiment 1
[0029] see figure 1 , figure 1 A schematic diagram of the steps of a similarity image retrieval method based on sparse coding provided by an embodiment of the present invention, which includes the following steps:
[0030] Step S100, performing basis vector characterization according to the reference image to obtain a first sparse characterization result;
[0031] Specifically, the reference image is the selected image to be retrieved, and the base vector is the most basic component in the vector space, because the base vector must be linearly independent, and this part can represent each vector in the vector space, reducing the dimension of the image, Obtain the first sparse representation result.
[0032] In some implementation manners, the electronic device shoots in various scenes, such as night scenes or backlit environments. In the same shooting scene, the electronic device can capture multiple frames of images, and perform image registration on the multiple frames of...
Embodiment 2
[0047] see figure 2 , figure 2 A schematic diagram of detailed steps of a similarity image retrieval method based on sparse coding provided by an embodiment of the present invention, which includes the following steps:
[0048] Step S200, fixing the basis vectors in the dictionary and adjusting the encoding coefficients to minimize the objective function;
[0049] Specifically, the dictionary training is completed by keeping the base vectors in the dictionary unchanged and changing the coding coefficients to minimize the objective function.
[0050] Step S210, fixing the encoding coefficients, adjusting the basis vectors in the dictionary to minimize the objective function;
[0051] Specifically, by keeping the coding coefficients unchanged and changing the basis vectors in the dictionary, the objective function is minimized, thereby completing the dictionary training.
[0052] Step S220, by continuously iterating until convergence, a set of basis vectors that well expres...
Embodiment 3
[0074] see image 3 , image 3 It is a schematic diagram of modules of a similarity image retrieval system based on sparse coding provided by an embodiment of the present invention. A similarity image retrieval system based on sparse coding, which includes a first acquisition module, which is used to perform base vector representation according to a reference image to obtain a first sparse representation result, and a second acquisition module, which is used to perform base vector representation according to an image in an image database The second sparse characterization result is obtained by vector characterization, the calculation module is used to calculate the similarity between the first sparse characterization result and the second sparse characterization result, and the judgment module is used to judge whether the similarity is greater than a preset threshold, and if so, the judgment is yes Similarity image, if not, it is judged as a non-similarity image.
[0075] It...
PUM
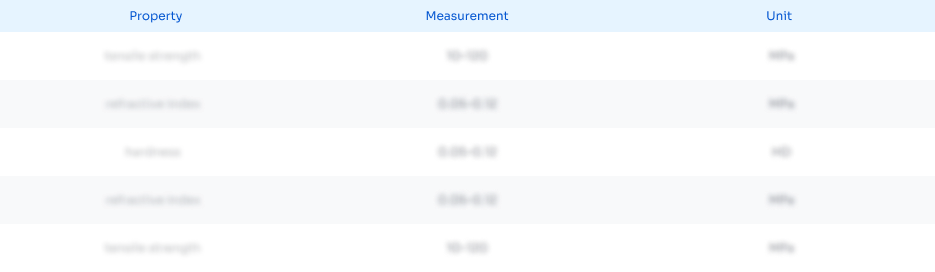
Abstract
Description
Claims
Application Information
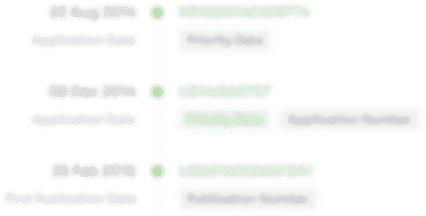
- Generate Ideas
- Intellectual Property
- Life Sciences
- Materials
- Tech Scout
- Unparalleled Data Quality
- Higher Quality Content
- 60% Fewer Hallucinations
Browse by: Latest US Patents, China's latest patents, Technical Efficacy Thesaurus, Application Domain, Technology Topic, Popular Technical Reports.
© 2025 PatSnap. All rights reserved.Legal|Privacy policy|Modern Slavery Act Transparency Statement|Sitemap|About US| Contact US: help@patsnap.com