A Defensive Agent Approach to Improve Byzantine Robustness of Distributed Learning Systems
A Byzantine robustness and learning system technology, which is applied in the field of defensive agents to improve the Byzantine robustness of distributed learning systems, can solve problems such as disrupting the normal training process of classifiers, parameter update decision errors, and defense mechanism failures, etc., to achieve robustness. Improvement of stickiness, relief of impact, and noticeable effect
- Summary
- Abstract
- Description
- Claims
- Application Information
AI Technical Summary
Problems solved by technology
Method used
Image
Examples
Embodiment Construction
[0032] Such as figure 1 As shown, the present embodiment adopts the CIFAR-10 data set, and the method of the present embodiment promotes the classifier as a distributed learning with 50 working nodes of the deep convolutional neural network ResNet-18 for object recognition (very class task) Byzantine robustness of the system training process. This embodiment specifically includes:
[0033] Step 1. Initialization phase: Before the distributed system training starts, prepare the private small verification set on the master node and initialize the credibility vector of the adaptive credibility evaluation module.
[0034] (1.1) Prepare a small verification set: uniformly sample K random data samples from the CIFAR-10 training data set as a small verification set S. In this embodiment, the small verification set size K is selected as 10.
[0035] (1.2) Credibility vector initialization: initialize the credibility vector of the zero-time adaptive credibility evaluation module ...
PUM
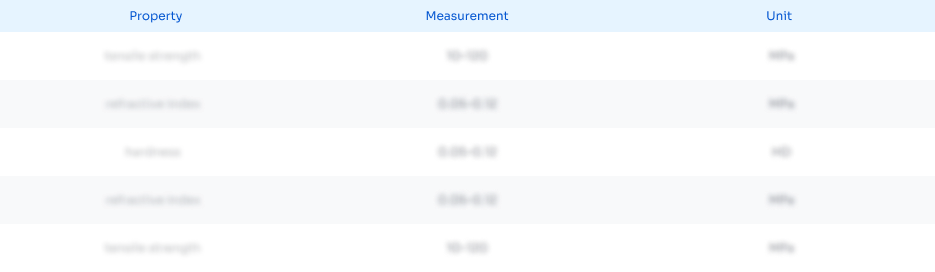
Abstract
Description
Claims
Application Information
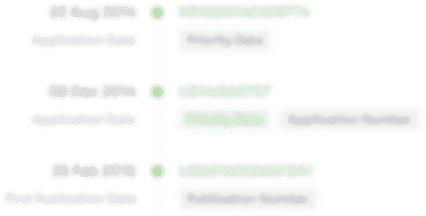
- R&D
- Intellectual Property
- Life Sciences
- Materials
- Tech Scout
- Unparalleled Data Quality
- Higher Quality Content
- 60% Fewer Hallucinations
Browse by: Latest US Patents, China's latest patents, Technical Efficacy Thesaurus, Application Domain, Technology Topic, Popular Technical Reports.
© 2025 PatSnap. All rights reserved.Legal|Privacy policy|Modern Slavery Act Transparency Statement|Sitemap|About US| Contact US: help@patsnap.com