Improved YOLOv3 model side-scan sonar shipwreck target automatic identification method based on transfer learning
A technology of transfer learning and side scan sonar, applied in the field of side scan sonar image target recognition and deep learning, can solve the problems of high false alarm rate, slow recognition speed, poor FasterR-CNN model effect, etc., to reduce leakage The effect of alarm rate, preventing over-fitting and rich detection efficiency
- Summary
- Abstract
- Description
- Claims
- Application Information
AI Technical Summary
Problems solved by technology
Method used
Image
Examples
Embodiment Construction
[0039] Below the experiment of the present invention is described in detail in conjunction with accompanying drawing:
[0040] The training and testing of this experiment are all based on the TensorFlow framework using python programming. The experimental environment is: Linux: Ubuntu18.04 version operating system; the CPU is Inter(R) Xeon(R) CPU E5-2678 v3@2.50GHz; the GPU is NVIDIA TITAN RTX, 24GB memory.
[0041] This paper is based on the YOLOv3 model, and the specific model structure is as follows figure 2 As shown, the YOLOv3 model uses the Darknet-53 network structure for image feature extraction. As shown in the figure, the network is mainly composed of 53 1×1 and 3×3 convolutional layers (Convolutional), which are located before the Res layer. And each convolutional layer will be followed by a BN layer and a LeakyReLU layer, which together form a DBL, such as figure 2 Shown are the basic components of the YOLOv3 network structure. The YOLOv3 model adds a skip con...
PUM
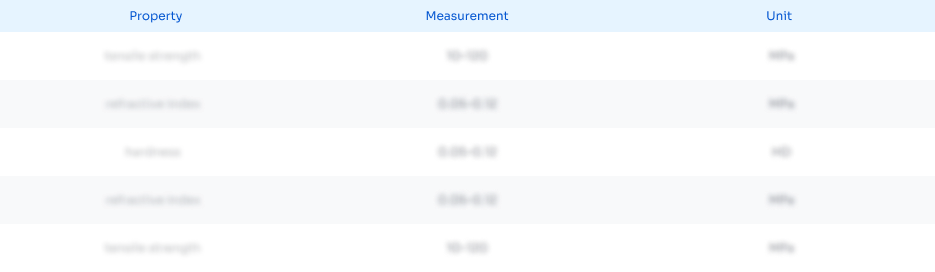
Abstract
Description
Claims
Application Information
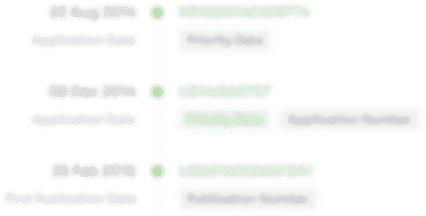
- R&D Engineer
- R&D Manager
- IP Professional
- Industry Leading Data Capabilities
- Powerful AI technology
- Patent DNA Extraction
Browse by: Latest US Patents, China's latest patents, Technical Efficacy Thesaurus, Application Domain, Technology Topic, Popular Technical Reports.
© 2024 PatSnap. All rights reserved.Legal|Privacy policy|Modern Slavery Act Transparency Statement|Sitemap|About US| Contact US: help@patsnap.com