Cross-correlation collaborative filtering method fusing weak trace behavior preferences of user
A collaborative filtering and cross-correlation technology, applied in the field of cross-correlation collaborative filtering that integrates users' weak trace behavior preferences, can solve problems such as difficulty, calculation deviation of user preference similarity, and different scoring scales, so as to improve accuracy and effectiveness , reduce sparsity, improve the effect of accuracy
- Summary
- Abstract
- Description
- Claims
- Application Information
AI Technical Summary
Problems solved by technology
Method used
Image
Examples
Embodiment Construction
[0048] Below in conjunction with accompanying drawing, the present invention will be further explained;
[0049] Such as figure 1 As shown, taking e-commerce product recommendation as an example, a cross-correlation collaborative filtering method that integrates user weak trace behavior preferences specifically includes the following steps:
[0050] Step 1. Construct the user's rating matrix and weak trace behavior matrix:
[0051] Assuming that the number of users and products are n and m respectively, and the score interval of the rating value is [0,5], the user weak trace behavior matrix B and rating matrix R are established o , the size of the matrix is n*m:
[0052] b i,j =(x|x∈{0,1}) (1)
[0053] r i,j =(x|x∈[0,5]) (2)
[0054] Determine whether user i has a weak trace behavior for product j by whether the click occurs and the time when the product is browsed, and the result is represented by b i,j said, b i,j =0 means no behavior, b i,j = 1 means that a weak tr...
PUM
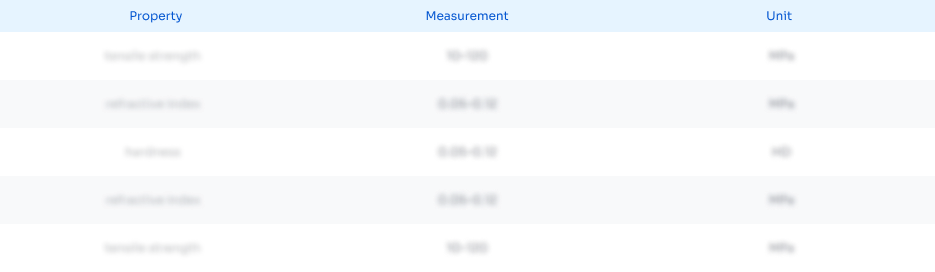
Abstract
Description
Claims
Application Information
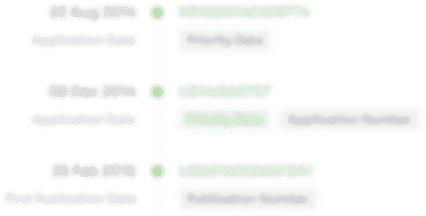
- R&D Engineer
- R&D Manager
- IP Professional
- Industry Leading Data Capabilities
- Powerful AI technology
- Patent DNA Extraction
Browse by: Latest US Patents, China's latest patents, Technical Efficacy Thesaurus, Application Domain, Technology Topic, Popular Technical Reports.
© 2024 PatSnap. All rights reserved.Legal|Privacy policy|Modern Slavery Act Transparency Statement|Sitemap|About US| Contact US: help@patsnap.com