Gini exponent-based domain adaptive semantic segmentation method
A Gini index and semantic segmentation technology, applied in the field of pattern recognition and computer vision, can solve a lot of experimental noise and other problems, and achieve the effect of improving accuracy
- Summary
- Abstract
- Description
- Claims
- Application Information
AI Technical Summary
Problems solved by technology
Method used
Image
Examples
Embodiment
[0122] 1. Experimental data set
[0123] The method proposed in the present invention is tested on the commonly used unsupervised adaptive dataset GTA5-Cityscapes, where the synthetic dataset GTA5 is used as the source domain and the real dataset Cityscapes is used as the target domain. Models are evaluated on the Cityscapes validation set.
[0124] GTA5: The synthetic dataset GTA5 contains 24966 synthetic images with a resolution of 1914×1052 and the corresponding ground-truth. These composite images were collected from a cityscape video game based on the city of Los Angeles. The automatically generated ground-truth contains 33 categories. The methods experimented on GTA5-Cityscapes generally only consider the 19 categories compatible with the Cityscapes dataset, and the present invention is no exception.
[0125] Cityscapes: As a dataset collected from the real world, Cityscapes provides 3975 images with fine segmentation annotations. The training set contains 2975 image...
PUM
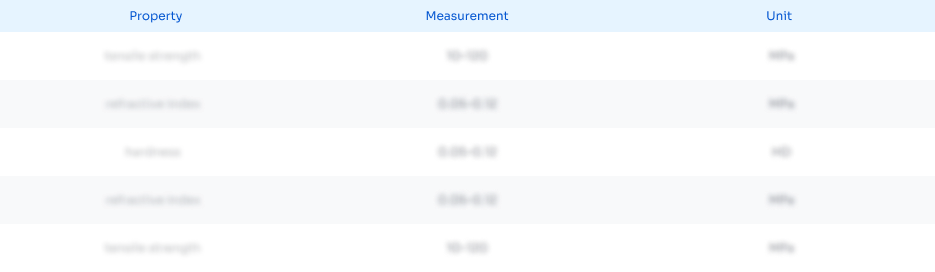
Abstract
Description
Claims
Application Information
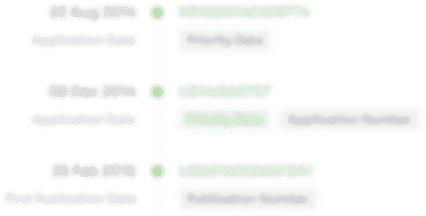
- R&D Engineer
- R&D Manager
- IP Professional
- Industry Leading Data Capabilities
- Powerful AI technology
- Patent DNA Extraction
Browse by: Latest US Patents, China's latest patents, Technical Efficacy Thesaurus, Application Domain, Technology Topic, Popular Technical Reports.
© 2024 PatSnap. All rights reserved.Legal|Privacy policy|Modern Slavery Act Transparency Statement|Sitemap|About US| Contact US: help@patsnap.com