Video depth relation analysis method based on multi-modal feature fusion
A feature fusion and relationship analysis technology, applied in the field of computer vision, can solve problems such as inability to solve long video deep relationship analysis, difficulty in combining short video analysis, and inability to solve multiple entities, etc.
- Summary
- Abstract
- Description
- Claims
- Application Information
AI Technical Summary
Problems solved by technology
Method used
Image
Examples
Embodiment Construction
[0027] The present invention proposes a video depth relationship analysis method based on multimodal feature fusion, and establishes a multimodal feature fusion network for identifying entity relationship diagrams in videos, such as figure 1 As shown, the network input includes video, scene screenshots and scene names and character screenshots and character names, and the output is a relationship diagram between the corresponding scenes and characters. The output is used for the problem types of long video analysis. Figure to answer. The input of the multimodal feature fusion network is given by the dataset during the training phase and manually during the test usage phase. The realization of the multi-modal feature fusion network is as follows: first, the input video is divided into multiple segments according to the scene, vision and sound models, each segment is an act, and sound and text features are extracted in each act as the act features , and then identify the locati...
PUM
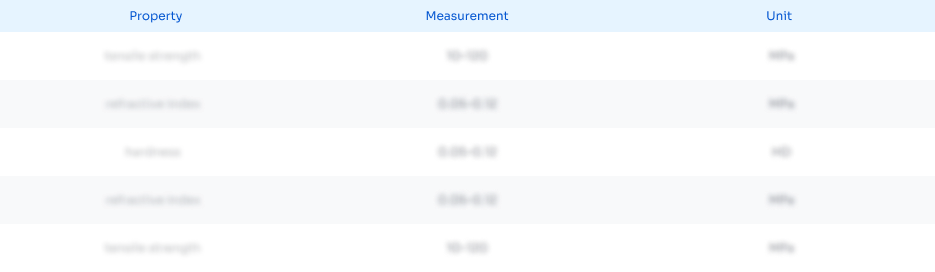
Abstract
Description
Claims
Application Information
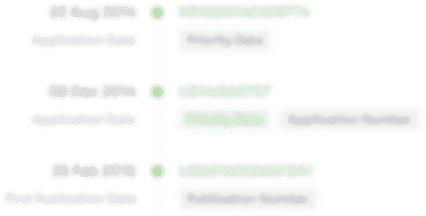
- R&D Engineer
- R&D Manager
- IP Professional
- Industry Leading Data Capabilities
- Powerful AI technology
- Patent DNA Extraction
Browse by: Latest US Patents, China's latest patents, Technical Efficacy Thesaurus, Application Domain, Technology Topic, Popular Technical Reports.
© 2024 PatSnap. All rights reserved.Legal|Privacy policy|Modern Slavery Act Transparency Statement|Sitemap|About US| Contact US: help@patsnap.com